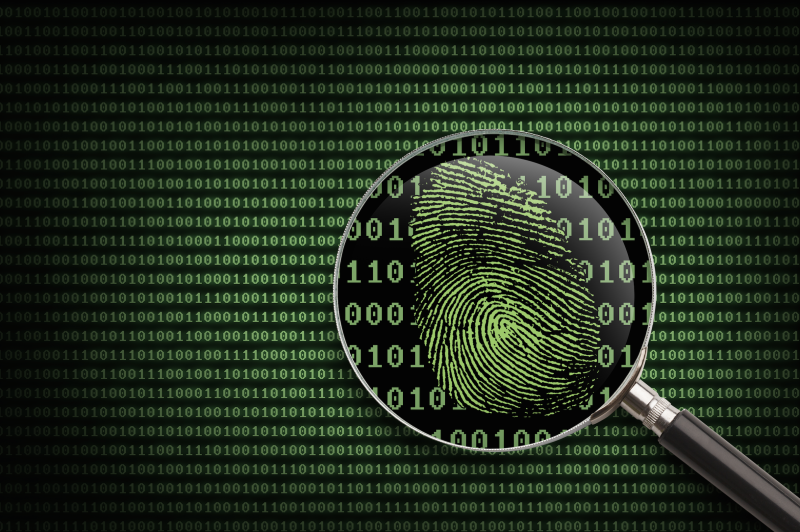
The topic of advertising fraud in the programmatic sector is a jugular issue concerning marketers today. The openness that allows advertisers and publishers of any size to participate in the programmatic ecosystem also lets bad actors participate and pollute the quality of the sector.
Fraud is the first thing that a marketer must address when looking at the overall quality of their ad campaigns. It is the fundamental first step before trying to optimize for viewability or brand safety, because it essentially poisons everything else if left unchecked.
With respect to sizing the impact of ad fraud, this excerpt from AdExchanger provides a guesstimate:
The Association of National Advertisers (ANA) and ad fraud solutions provider White Ops this month collaborated on a 60-day study looking at the severity of bots. The study tracked 181 campaigns among 36 ANA members (including Walmart, Johnson & Johnson and Kimberly-Clark) and determined that bots cause 23% of all video impressions, 11% of display ads and would account for $6.3 billion in losses in 2015.
Industry research pegs it quite high, but that is looking at this space in aggregate.
Based on data we see on our company’s platform, fraud varies greatly by ad exchange. Exchanges with poor reputations for quality have fraud in the 25–50 percent range, whereas reputable exchanges typically have less than 10 percent.
Most of the industry discussion around fraud, however, only scratches the surface in a superficial way and commonly conflates different issues under the broad label of “fraud.” Many discussions of fraud explain it in terms that are too simplistic and fail to underscore the nuances of this issue.
This piece focuses on the definition of fraud and the various ways it takes shape.
Defining Fraud
It’s surprising how much disagreement and confusion there is around defining ad fraud. One of the biggest mistakes I see is the blanket definition of ad fraud as non-human (i.e., bot) traffic.
While that is certainly a characteristic of some forms of ad fraud, to make it a criterion for defining fraud is to miss the bigger picture: A significant percentage of fraud is actually human traffic, as we will soon explore.
Another way of thinking about this logic is as follows: While all bot traffic is indeed fraud, not all fraud is bot traffic — just like fire is indeed hot, but not all hot things are fire.
With that in mind, let’s look at a refined definition. Ad fraud has one or more of these characteristics:
• Non-human traffic (i.e., bots).
• Zero chance of being seen (i.e., zero percent viewability).
• Intentionally misrepresented.
Most anti-fraud efforts, and most press coverage, seem to focus solely on non-human traffic. But fraud goes far beyond bots. It also includes ads that have zero chance of being seen by a human and ads that are intentionally misrepresented by publishers.
Let’s begin by briefly covering each of these characteristics, starting with the non-human traffic.
Non-Human Traffic
Most non-human traffic is used to generate fake impressions (page views) and fake clicks. In some cases, they go so far as to generate fake form submissions, and therefore, fake conversions.
• Simple Bots: Simple bots are essentially just scripts that run from a server somewhere, like Amazon Web Services or some other hosting provider. Because they are simple, they are usually easy to identify — since they have a static IP, user agent, cookie ID and so on — which makes fingerprinting and blocking them relatively easy.
A simple look at some DSP auction logs, or even Web server logs from those that click through, make simple bots fairly easy to detect and block. For example, one could simply block all known data center IPs.
• Sophisticated Bots: Sophisticated bots, on the other hand, employ tactics like rotating user agents, using random proxies (to rotate IP addresses), mimicking normal click-through rates, and in some cases, even mimicking real mouse movements from captured browser activity. All of these factors make it harder to fingerprint and block them.
• Botnets: Botnets are generally a large array of personal (residential) computers that have been compromised by bad actors. These actors have control of these machines, employing them for tasks like loading and clicking on ads, which generates legitimate-looking, but ultimately fake, impressions and clicks for advertisers.
Botnets are the hardest to detect and block, but they are also highly illegal and, therefore, riskier for bad actors to deploy. (To see an actual demonstration of how an infected computer behaves as a bot, check out this eye-opening video from the folks at Integral Ad Science.)
Nevertheless, since bots are programmable, they often exhibit patterns that make them detectable by good experts. They also make for more enticing headlines, so they typically receive the lion’s share of media coverage.
Human Traffic
Human traffic, on the other hand, is perhaps even more sinister because the end users are real, but the impressions (and in some cases, clicks) generated are fraudulent. Since they are real people, it can be harder to detect by vendors that are only looking for bots.
• Invisible Ads: There are a few common ways that fraudulent publishers “hide” ads so that they fit the criterion of having “zero chance of being seen” by a human visitor.
The first is referred to as “ad stacking” or “impression stacking,” which is basically hiding ads behind other ads. In such cases, the publisher is generating multiple impressions for a single page view, but the only ad that is visible is the top one.
In a similar vein, invisible iFrames are another way of intentionally hiding ads. By loading ads in unviewable (1 pixel by 1 pixel) iFrames, an impression or multiple impressions are generated, with no chance of ever being seen. Such tactics are relatively easy to detect using off-the-shelf ad verification tools like Integral Ad Science or Pixalate.
• Arbitrage: One of the most under-reported but insidious forms of human-based traffic fraud is a form of arbitrage. It can take many shapes and affect multiple formats, such as display and, most notably, video.
In essence, the bad actors purchase traffic for a very low cost and resell it for a multiple of the price. For example, a publisher may sell their inventory for $5 CPM on average but may be purchasing questionable traffic to their site for a fraction of that cost.
• Domain Spoofing: In the RTB (real-time bidding) ecosystem, publishers are sometimes allowed to declare their own domain and the label of their Site ID. Fraudulent publishers use this as an opportunity to misrepresent their inventory. They may identify themselves as huffingtonpost.com, but if you dig deeper, the actual domain the ad was served on was different. In other cases, the ad-serving domain is spoofed within the bid request.
• Site Bundling: Site IDs are how inventory is classified in the RTB ecosystem. The way RTB was designed, each Site ID was supposed to correlate to a single domain.
But in practice, many publishers and exchanges bundle entire networks of domains under single Site IDs. So an advertiser might think they are buying abc.com but end up with ads served on xyz.com.
This configuration happens on the supply side, which is outside the control of DSPs (demand-side platforms). This falls under the “intentionally misrepresented” inventory category.
• Ad Injection: As a result of browser toolbars and other adware plugins, ads can get injected and/or replaced on any site, often without the user or publisher noticing. This creates a situation where ad inventory might show up as facebook.com, for example, but is no way related to Facebook’s actual ad inventory.
After all, any Facebook user could tell you that there are no 300×250 or 728×90 banner placements. But with ad injection, inventory can be created on premium websites out of thin air.
• Cookie Stuffing: The practice of cookie stuffing is nothing new to the world of online advertising. There have been some high-profile cases of cookie stuffing where it was used to maximize affiliate revenue.
Cookies are just as important today, because they are the mechanism through which a large part of the programmatic ecosystem targets audiences. And with inexpensive sources of internet traffic available for purchase, cookie stuffing to dilute or misrepresent audience target data is now a real thing.
It’s also worth noting that cookie stuffing can occur on both human and non-human traffic, so technically, it could fall into either category, and sometimes both.
• Click Farms: There are incentivized programs, often masked as “work from home” or “make money online” schemes, that pay real people to click on ads and even fill out forms, resulting in valueless impressions, clicks and conversions.
Since these are real people, it’s hard for most software vendors to catch these schemes, but they are most definitely fraudulent activity. (Check out this video to enjoy a good parody of what these schemes look like.)
Looking Forward
Now that we have a better understanding of the different types of ad fraud, and the nuances between them, it raises some very important questions:
• Why does ad fraud exist in the first place?
• Who is responsible for ad fraud?
• How do marketers protect themselves against fraud?
We will answer all these questions and more in subsequent articles. Also, if this topic interests you, I will be speaking at ad:tech NYC on November 4 on “Understanding The Many Faces of Ad Fraud (And How To Protect Yourself).”
During that presentation, we will go much deeper into why fraud exists, who is responsible for it, how to deconstruct fraudulent publishers and best practices for protecting yourself as a marketer.
(Special thanks to Ian Trider at Centro, and Ian Johnson at Cadreon, for their helpful insights.)
Comments