A Concise Overview Of Cross-Channel Marketing Models
- Fahad H
- Apr 17, 2014
- 3 min read
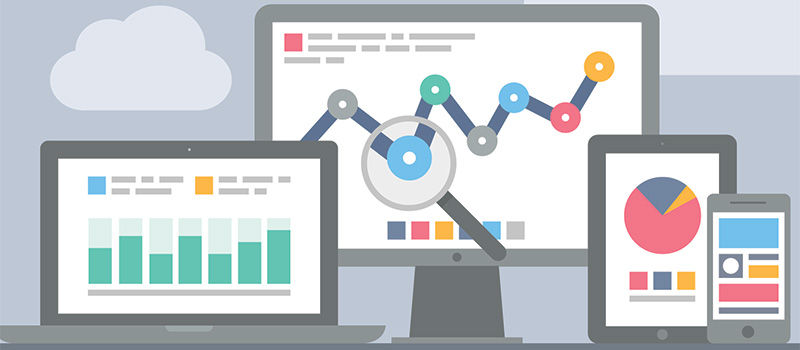
For several decades, modeling cross-channel marketing impact was synonymous with marketing mix (“top-down”) models. However, with the increase in cross-channel tracking and computational power, new breeds of marketing attribution (“bottom-up”) and other alternative models have appeared in recent years.
Although these models all seek to quantify the relative impact of different media channels, they are quite different in their approach.
And while there are some articles comparing these different methods, I have yet to find a free unbiased resource that gives an overview of the subject; so, this is my attempt to fill the gap.
This overview intentionally avoids evaluation of the approaches listed. Each method has its own strengths and weaknesses, and the choice of which to use will depend on the particular need of the organization.
Common Approaches to Modeling Marketing Impact
The most frequently applied methods for modeling cross-channel marketing impact today are the following:
Econometric top-down approach (e.g., marketing mix models)
Algorithmic bottom-up approach (e.g., attribution models)
Machine learning approach (e.g., agent-based models)
Below, I will give a high-level summary of what each approach involves and their respective strengths and weaknesses.
Econometric Approach
The classical econometric approach to marketing mix has the longest history of practice and is widely applied today. Commonly used for informing high-level budget allocation, this approach typically employs some form of distributed lag regression to estimate the impact of marketing activity on total sales.
Traditionally performed as individual consulting projects, some vendors have been working to automate and productize this method.
Outline of approach:
Compile historical spend and marketing activity data across media channels, along with conversion/sales data, and exogenous (non-media) factors such as economy, pricing changes, etc.
Build a statistical model to assess the effect of these variables on sales or other KPI
Quantify the impact of each marketing channel or campaign
Strength: Data-driven statistical approach allows for high predictive capability.
Weakness: Granularity of insights and what-if scenarios are conditional on granularity of input data.
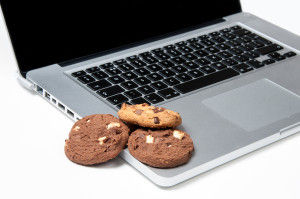
Algorithmic Approach

The touchpoint based approach to marketing attribution was made possible only recently via cross-channel tracking. These models are unique in that it looks at cookie-level event data, and attempts to determine the impact of each type of touchpoint from sequence patterns and results.
Over the past several years, a number of vendors have offered up marketing attribution solutions, either the bottom-up approach alone or in conjunction with a top-down approach.
Outline of approach:
Track cookie-level data on marketing touchpoint sequences along with conversion activity
Compare conversion rate for a sequence to similar sequences in order to assess effect of a particular touchpoint being present
Aggregate results across sequences to assign relative value to media or campaign types
Strength: Touchpoint-level calculation allows for very granular attribution and impact assessment for tracked media.
Weakness: More descriptive than predictive; cannot by itself incorporate offline or non-tracked online media.
Note: I will not cover rules-based allocations such as even distribution or U-shaped here. Here are some reads on the rules-based vs. algorithmic attribution.
Machine Learning Approach
Agent-based models treat individuals as “agents” that interact with events or stimuli to exhibit certain behaviors, and utilizes computational simulations to assess impact.
The concept of agent-based modeling first appeared in the 70’s, gaining adoption in social sciences in the 90’s. Application toward the field of marketing has been delayed due to the computational complexity involved, but recent years have seen attempts to apply this approach to marketing impact modeling.
Outline of approach:
Create a simulated population for the business using demographic data and understanding of customer profile
Train and calibrate the simulated population using historical marketing activity, along with conversion/sales data and exogenous factors
Simulate reaction to various marketing activities and changes
Strength: Individual-based models allow for flexible simulations at customer demographic level.
Weakness: Accuracy of results is only as valid as the behavioral assumptions for the business and demographic segment.
Future of Marketing Impact Assessment
As marketing enters an era of accountability, the demand for cross-channel marketing impact assessment will continue to increase. The next decade will see further refinement and combination of these approaches to modeling marketing impact. We already see this trend in the market, with some vendors combining top-down and bottom-up approaches.
In reality, however, there is no such thing as a perfect model. It is impossible to account for all the variables that may impact business or marketing performance, and some – such as a viral marketing success or stock market crash – are virtually impossible to predict.
After baseline model accuracy and complexity reaches a plateau, these unpredictable or unaccounted-for factors will play a large role in determining how accurate and useful the models are in practice. As a result, the key differentiator is likely to become real-time simulation and prescriptive capabilities:
Support of predictive what-if scenarios at granular cross-sections (e.g., brands, products, markets, segments) and levels (e.g., campaign, publisher, target, creative)
Prescriptive functionality and workflow that allows marketers to take immediate action based on the most recent trends and campaign results
Images via Shutterstock.com, used by permission.
Comments