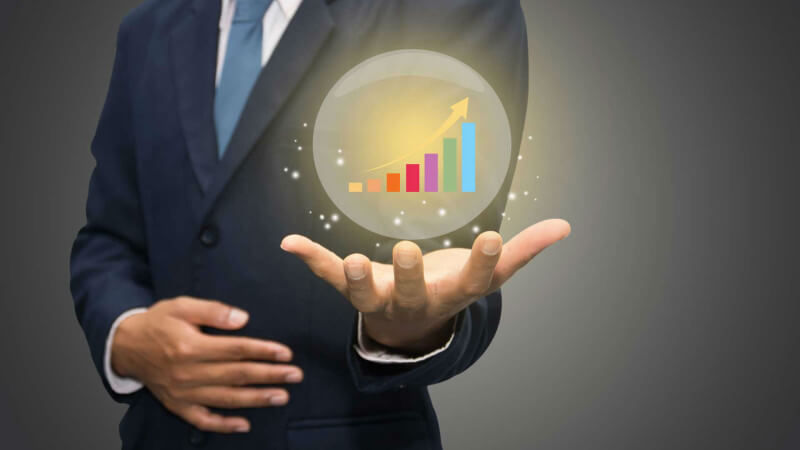
If you’re already using predictive analytics within your organization, then you know just how impactful they can be, and you’re likely on the lookout for new and different applications to drive your business even further.
And if you’ve ever been intrigued by the notion of using predictive analytics but thought you weren’t big enough, sophisticated enough, or just plain not ready for it, then keep reading: odds are pretty good these days that the benefits of predictive analytics are within your reach.
In this article, I’ll share three cases outlining ways to use your data to predict value and take your marketing initiatives to another level.
What is predictive analytics, anyway?
Predictive analytics is nothing new; indeed, it’s been around for a long, long time. What makes it particularly exciting these days is that never before in the history of marketing have we had so much data and so much processing power. This means we’ve got lots and lots of signals to play with to predict lots and lots of outcomes, and we can do it at higher speeds and lower costs than ever before.
Generally, predictive analytics is just a way to help identify the probability of future outcomes based upon historical data. From the customer perspective, you can use it to predict a likely lifetime customer value or the probability of either loyalty or churn. With respect to a marketing budget, you can use predictive techniques to gain insight into the best ways to allocate your media mix or understand the likely effectiveness of a potential campaign.
Operationally, predictive analytics can help you forecast a host of elements across many of your business functions to enable you to plan and budget more effectively, and predictive has long been used in understanding potential risk, ranging from collections to fraud detection.
Here, though, we’re going to focus on some examples specific to the digital marketing space.
1. Optimizing digital spend toward actions that are highly predictive of future revenue
We all know that there’s a path to purchase, and it usually doesn’t start with the purchase itself. That means there are lots and lots of touch points out there, and in the digital world, we’re capturing data around just about all of them.
So wouldn’t it be great to understand the value of each of those touch points and digital actions? Or to be able to predict just which types of products and services those touch points are likely to be leading toward?
Let’s take an example of a client in the auto aftermarket industry that we worked with at my company, Cardinal Path. This is a great example, particularly because despite having an extremely large marketing spend across a host of digital channels, there’s not a single thing you can purchase online. And in order to have your car serviced by this organization, you’re going to have to physically go to a location and participate in an offline transaction.
So how can this enormous gap between online advertising and offline purchases be bridged? With data. The traditional approach was what many marketers are still doing. They would look at things they could drive in the digital world — things like click-to-call actions or usage of a store locator function — and assume that these were the kinds of things that would drive people to an offline interaction.
And while logically, this makes a lot of sense, it’s really hard to assign an actual value to those actions in order to guide a media strategy focused on performance, returns on marketing dollars, and ultimately, a cost per action (CPA).
But by leveraging all the in-store and transactional data and merging it with the enormous amount of digital data, a model can be built to help identify just what digital touch points are likely to lead to which offline purchases.
The result is that marketers no longer have to “kind of maybe try to hit a CPA around, maybe, I don’t know, ten bucks or so?” and instead, can predict the actual value of each particular action in each product category (sample summary with dummy data below), giving some very tangible and actionable goal posts for everyone involved in planning and buying digital media.

By using predictive analytics to look at purchase intent, we found some pretty interesting things. First, we were, of course, able to validate and put numbers around some of those assumptions. If someone was in a situation with an urgent need, like a flat tire or a dead battery, then we found that signals like click-to-call and use of a store locator function were indeed very predictive of an imminent purchase of those types of product sets.
But we also found that signing up for an email newsletter was highly predictive of some type of regular maintenance in the near term. Why? Well, consumers tend to have the expectation that signing up for a newsletter means a significant coupon shortly after joining. And we also saw that applying for financing through a branded credit card meant that the old “check engine” light wasn’t going away any time soon, and a big-ticket item or repair was on the horizon.
2. Leveraging a testing plan against high-value outcomes
Continuing down this path, it’s easy to see that if we’re able to predict just what certain online touch points and actions are likely to be worth, we can optimize not only our digital marketing campaigns, but also our testing plans.
It’s getting pretty tough to find a digital marketer that hasn’t discovered the magic of conversion rate optimization and structured testing, and organizations are using a host of tools and technologies to design, plan, implement and analyze all kinds of tests ranging from simple A/B to complex multivariate experiments.
But while the methodologies and supporting tech continue to reach even higher levels of maturity, there’s one basic issue that continues to plague testing programs everywhere: What exactly should we be testing?
Enter predictive analytics, and specifically, another use for purchase intent. We’ve been fortunate to work with US Cellular on a number of initiatives, and recently, we set out on a project to answer two key questions:
What exactly is the role of website actions in driving in-store sales?
How can we use this information to “optimize what we optimize?”
Think about the last time you purchased a mobile phone. Consumers tend to prefer to do lots of their pre-purchase research online, but they also prefer the instant gratification of holding the new device, transferring all their photos and contacts and walking out of a store or away from a kiosk with the ability to immediately use that new device.
So once again, applying predictive analytics on a range of digital and offline data sets, we were able to identify just how valuable different online behaviors were to an offline, in-store transaction and activation later in the purchase cycle.
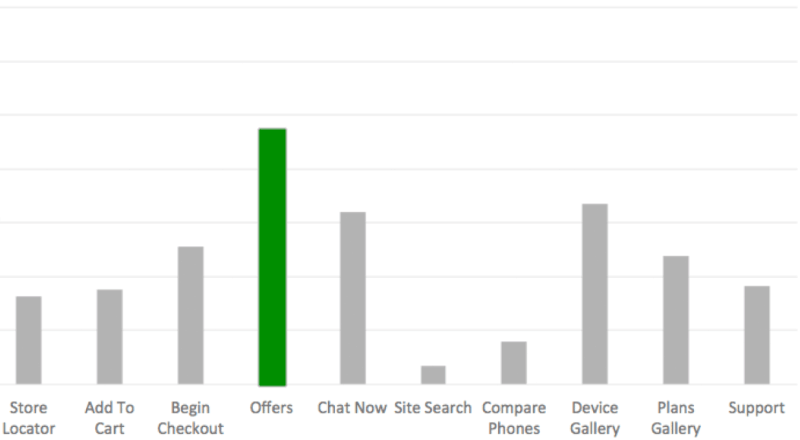
The data told a story with many elements we might have expected: Add-to-cart actions and beginning a checkout process were indeed predictive of an impending offline purchase, and locating a nearby store also showed up as an action predictive of purchase intent. But browsing device galleries and using the chat feature were among the more valuable actions, and the single most important factor in purchase intent was interacting with the current special offers.
So what can we do with this information? Going back to the objectives of this initiative, we can use it to “optimize what we optimize” by prioritizing and focusing testing plans on the objective of getting users to interact with offers, an activity that we now know to be highly predictive of offline value.
And in the case of US Cellular, that’s exactly what they did. Instead of arguing about what tests to run, or wasting time playing politics or debating the subjective, this team was off running tests they knew would be valuable, and after two cycles were driving 23 percent more visitors to offers interactions, translating to a 61-percent lift in visitor lifetime value.
3. Predicting incremental campaign impact and understanding where NOT to spend
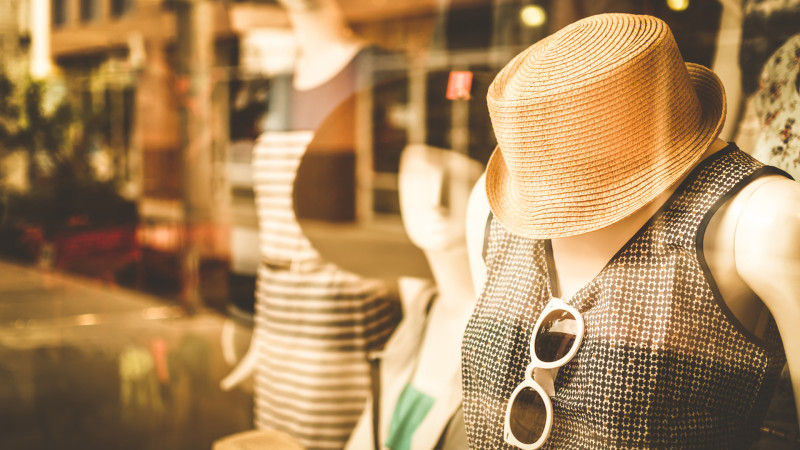
Predictive analytics can also help determine just which campaigns are most valuable in driving actual sales. Of course, attribution and media mix modeling are essential tools for this, but there’s plenty of low-hanging fruit to pick in understanding the relationship between customer segments and the marketing campaigns they’re interacting with.
In working with a global, fast-growing fashion retail group, we built models that could predict the probability of a particular channel influencing online or offline purchases within specific customer segments, and while this was enormously useful in understanding how to spend budgets targeting more personalized digital campaigns, it was equally insightful into identifying spend that wasn’t contributing to incremental value.
And this is a wonderful question to be able to answer: Should we pay for this ad, or will a sale happen organically through another channel or communication that might cost nothing or next to nothing?
The approach was very similar to other examples we’ve looked at. By introducing the various marketing channels, customer attributes and transaction data into a model, we were able to predict purchase behavior based on customer attributes and channel touch points. With this information, the digital strategy could then be transformed with the confidence of a data-driven approach.
First, this allowed the marketing team to choose the right channels to most effectively and efficiently reach different groups of prospects and customers, and second, it provided the information required to personalize by sending the right content and message to the right segments at a very granular level.
Go start predicting
While these examples did include some sizable organizations, and there are some thresholds with respect to the type and volume of data available, these weren’t the largest or most organized data sets around.
The truth is a tremendous amount of predictive analysis can very likely be done on whatever data you do have, and starting down or continuing to traverse this path will continue to uncover more opportunities for analysis that will lead to the collection and integration of more and more data over time.
But once you start, one thing is for certain: You’ll be using your data to drive your decision making, and that glimpse into the future will reap some very real rewards.
Kommentare