KPI Frameworks: Bringing Order To KPI Chaos To Prioritize E-Commerce Optimization Efforts
- Fahad H
- Nov 9, 2015
- 4 min read
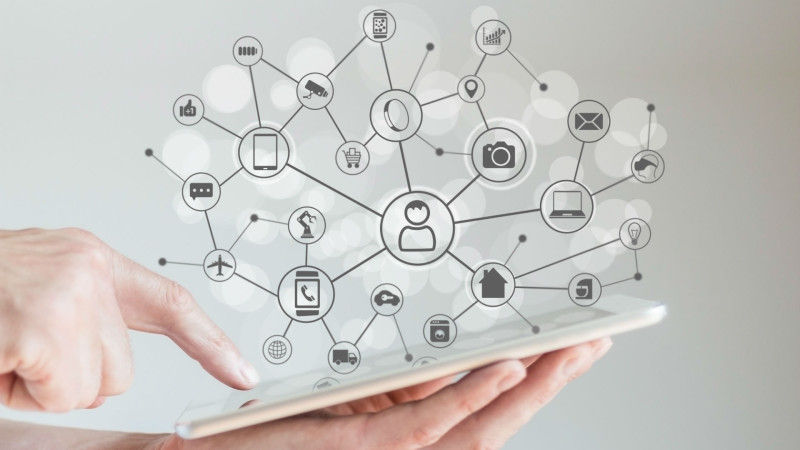
By now, it’s cliché, but true: analysts are drowning in data when it comes to e-commerce.
On the one hand, it’s great to work in an industry where there’s so much data which you can potentially put to valuable use. On the other hand, until you’re able to consistently sift through it and find the most actionable bits, all your data represents is potential.
Having so much data at your fingertips can actually prevent you from focusing on your true KPIs — the ones that are, quite literally, indicative of business performance.
For example: should e-commerce organizations be focused on optimizing against overall revenue? Average order value? Number of transactions from first-time buyers? Purchase frequency of repeat buyers? Some combination of the above? All of the above?
These are difficult questions for businesses to answer, in large part because different people within an organization are likely to have conflicting priorities. Ask your customer acquisition manager what the most important KPIs are, and you’re unlikely to get the same answer from your customer retention manager.
The explosion of data has changed the challenges businesses face when it comes to analytics. Technical implementation hurdles are much lower than they used to be; today, the bigger issue is bringing structure, context and clarity to your data.
This is a nice problem to have if you remember the dark days when simply getting reliable metrics from your website was a serious issue. Nevertheless, it is a problem — and one that prevents many businesses from going beyond collecting data to actually activating data to create value.
Establishing A Framework
To solve for this challenge, at Cardinal Path, we often help our clients develop what we call a “KPI Framework.”
A KPI framework groups related KPIs together, providing structure and context.
For instance, we often see clients measuring KPIs such as revenue, transactions, order size and all the other classic e-commerce KPIs. But we often find that they track these things in an ad hoc manner or in a vacuum, which is why we recommend grouping all their KPIs into a structured framework.
For instance, common frameworks focus on net income, or the idea of a customer lifecycle/journey. Lots of individual KPIs are needed to measure net income or an entire customer journey. By using these frameworks, related KPIs can be grouped together in a way that provides crucial context, helping you prioritize optimization efforts and understand how performance of certain KPIs impacts the performance of other KPIs.
Another common example is that while most clients are very good at tracking on-site conversion, many falter when it comes to tracking KPIs related to things like customer retention, satisfaction, and even advocacy.
The digital customer journey doesn’t end at conversion — you want to think about how to retain customers over time, since it’s generally much more efficient to keep existing customers than to go out and buy lots of new ones. So you may need to measure metrics like successful order fulfillment, average shipping time and more.
Similarly, you may want to measure how many customers go on to post positive reviews of your products, say nice things about your product or brand on social media, share their purchases with friends and so on.
In this scenario, you’ll still have a wide variety of KPIs to track, but because similar KPIs are grouped together, your respective teams will have real clarity on which metrics in fact measure their performance. Your customer retention team can focus on the KPI of purchase frequency for repeat buyers, while your social media people can focus on percentage of posts with positive sentiment. Meanwhile, executives can see how performance in one area (e.g., customer acquisition) has downstream impacts in other areas (e.g., total sales, customer retention).
Finding The Needle In The Haystack
KPI frameworks don’t just bring clarity to your teams as to which metrics they should be paying attention to. They also help you develop more specific hypotheses for optimization efforts. Without specific hypotheses based on relevant data, it’s hard to “move the needle” in a meaningful way.
For example, let’s say sales are flat, and you’re trying to figure out why.
You can check your retention KPIs and see that you’re actually performing better month over month. You know it’s not an issue with repeat customers, so now you’ve quickly narrowed your issue down to customer acquisition, pre-purchase engagement and so on.
Your basic traffic metrics show that you’re generating more sessions from more users than ever, meaning it’s not simply an issue of “filling your funnel” — so you cross that off, too.
Looking at your engagement KPIs, you notice that fewer people are signing up for your promotional emails, where you send out coupons and such. Now you’ve identified a lever you can pull that will actually make an impact on your “meta KPI,” which is sales.
Getting Beyond Implementation
We’re at a stage in the industry where the sheer implementation of an analytics tool is rarely the most pressing challenge in seeing positive return on your analytics investment. As implementation has gotten less challenging, the amount of data at our fingertips has ballooned.
It’s time to consider whether your analytics implementation is not only technically sound but truly driven by an agreed-upon KPI framework and business requirements.
If you want a higher “hit rate” on your optimization efforts — if you want analysts to come up with better, more specific, more relevant hypotheses — then don’t just worry about your technical tools or whether you have enough metrics to use. Start thinking about constructing a KPI framework for your business that helps you bring order to chaos.
留言