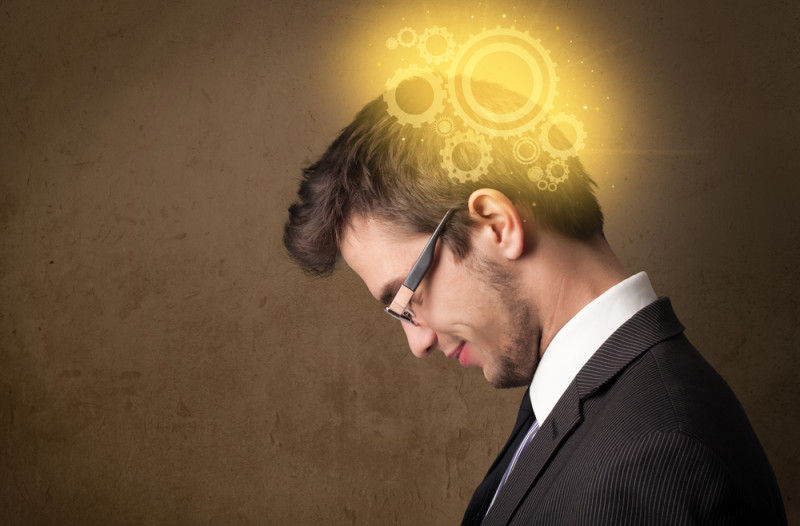
As marketing analytics continue to evolve at a breakneck pace, an interesting race is beginning to take shape: human intuition vs. machine-based intelligence.
Earlier this month, MIT researchers announced they’ve developed an algorithm that can trump human intuition. The “data vs. intuition” debate also popped up in multiple sessions at last month’s Advertising Week in New York, and it has been a recurring theme for years.
But in many ways, the conflict between human intuition and machine data is a false one. The real answer lies in changing the way humans interact with machines, and designing this new alliance is particularly important for marketers.
Intuition Is Data
There’s one interesting element of this “humans vs. machines” analytics discussion that isn’t addressed often, and that’s the fact that the human brain remains one of the most flexible and potent sources of computing on the planet.
Intuition is data. It’s just that human intuition is processed in the ultimate black box algorithm — the human brain — and none of us own the IP on that (at least not yet).
The human brain, uniquely designed to find meaning in shapes and visual patterns, engages with machine data through data visualization. Machine data, when it’s at its most effective, combines high-end computations with intuitive and engaging data visualizations.
And the most effective marketing analytics solutions are those that recognize that human intuition — and perhaps most importantly, human curiosity — plays a critical role in moving from data to insight to decision to action.
After all, human curiosity is nothing more than an organically data-driven impulse that something is worth further investigation. And human intuition is simply an organically data-driven conclusion that for reasons we may not be able to articulate, a correlation exists that we ought to try to understand further.
That’s why in many data scientist circles, including here at Quantifind (my employer), people are beginning to espouse the belief that rather than focusing solely on AI, or artificial intelligence, we ought to be focused on IA, or intelligence augmentation.
This allows us to go deeper with our intuition and expose our instincts to new inspirations. Data visualizations open up whole new worlds of possibilities for the human mind to react to and process.
In short, marketers should be looking for solutions that let them tap into their curiosity and instincts.
They should look for features that help them explore and understand their data better. They should be using both mind and machine to investigate the correlations that matter between what marketing is doing, what customers are saying and how their business is performing.
And through it all, they should seek to redesign the alliance between human intuition and machine-driven data. But to do so requires that we rethink what data does and doesn’t do.
Algorithms Are Tools, Not End-To-End Solutions
To be clear, the MIT research is great data science. Currently, humans are much better than machines at identifying promising patterns in large data sets.
That is, people define interesting problems, then use these definitions to create teaching sets for machine learning. This process has made algorithms a consequence of intuition.
MIT’s project, called the Data Science Machine, potentially changes this dynamic, identifying meaningful patterns without human guidance. In data science competitions, the MIT algorithm didn’t beat all human adversaries, but it beat most of them and worked exponentially faster.
Clearly, the research has a place. It will play a role in future advances and will expand the tasks that machines can be expected to reliably complete.
But like most technology, this sort of advance is a tool — good at some things, suspect at others.
To their credit, the MIT researchers recognize these limitations. “We view the Data Science Machine as a natural complement to human intelligence,” Max Kanter, whose MA thesis was the basis for the algorithm, told MIT News.
Marketers need to take a similarly measured approach. Neither data nor creative instinct alone is a full-fledged solution.
The key to success will be marrying the two approaches, which means that rather than buying into hype and treating data as omniscient, marketers need to recognize the limits of different methods.
The Data Science Machine, for example, currently works with relational databases — that is, the kind of structured data we commonly see in spreadsheets but not the unstructured, chaotic data generated by something like social media.
This sort of relational analysis has clear benefits and limits. The researchers illustrate that if a database contains beginning and end dates for various sales campaigns and weekly profits, “the crucial data may not be the dates themselves but the spans between them, or not the total profits but the averages across those spans.”
That’s true enough. Overall profit might be less important than some trend that causes short-term sales spikes, for example.
Identifying this trend and determining how to scale it across longer periods is more valuable than seeing cumulative figures. As a tool with strengths and weaknesses, the Data Science Machine could be ideal for this sort of work.
Unstructured data is different. Social media comments don’t fit into relation spreadsheets. They can’t be mined for meaningful correlations using the same techniques that work in a structured database.
For example, when movie theater websites crashed this month during the rush for Star Wars tickets, many commentators sarcastically commented on social media — e.g., “Fandango, load or load not, there is no try.” For an algorithm, it’s difficult to recognize that this sort of comment is a sarcastic criticism that could, if widely shared, have implications for the brand’s reputation.
For a person armed with intuition and experience, however, the relationship is obvious.
Human Curiosity And Creativity Will Always Matter
Creative interpretation of the data is always going to be essential. A marketer might use something like the Data Science Machine for some tasks while relying on a different set of algorithms and methodologies for other tasks.
When confronted with unstructured social data, for example, the marketer could correlate social comments to revenue. This would enable the marketer to identify consumer language patterns that correlate with sales growth and to tailor messaging to the themes expressed in these patterns.
But even then, this “tailoring” won’t be an automated process.
What’s more, only human instincts can help determine what questions are worth pursuing in the first place. The only thing worse than the wrong answer is the right answer to the wrong question. And only human discernment can tell the difference.
Finding a way to drive revenue that works only in theory is useless if it doesn’t also work politically, organizationally and psychologically for all the decision-makers involved.
Ultimately, then, marketers should ask themselves a few important questions as they approach their marketing data solutions:
What is the current relationship between my own subjective marketing instincts and my data solution? How do I want that relationship to change?
Who determines what questions I explore — the machine, me, or a combination of both? And who’s better positioned to know what questions lead to more revenue upside?
Where in the process have we accounted for political and organizational realities? Have we reverse-engineered our insights process to find answers that are not only valuable but also practically actionable?
Lastly, how does the data lead me to better, more fruitful questions to explore, and what room does this process leave for the serendipitous discovery of unexpected revenue opportunities?
Comentarios