How To Use Relative Standing For Online Analytics
- Fahad H
- Feb 21, 2014
- 6 min read
I was recently taking a fresh look at some old statistics school books of mine, and I realized how useful many of those basic statistical concepts can be when applied to online marketing analytics.
In this post, I’ll share a couple of ways you can delve into your data in a simple way by using relative standing — that is, how individual data values compare to the rest of the group, as opposed to straight absolute values, which are typically hard to put into perspective.
Getting Started: Mean Vs. Median
First off, if you are not familiar with statistics at all, let me briefly clarify the difference between the mean and the median; these statistics are two different ways to measure the center of the data:
The mean is simply the average value, so if we are looking into the revenue by keyword in paid search, that would be the total amount of revenue divided by the total number of keywords.
The median is the middle value in a data set after ordering the numbers from smallest to largest — or, if you have an even number of numbers in the data, the average of the two numbers in the middle of the data.
The median is often used because it gets less affected by outliers — those extremely low or high numbers in the data. Lots of statisticians argue that the median better reflects what the center of the data is.
Applied to online marketing, and more specifically to paid search, a single keyword with a super high CPA (cost-per-acquisition) could totally bias your mean CPA, while it would not bias your median CPA. Although the mean CPA is what you potentially mostly care about at the end of the day, the median CPA might be a better indicator of what your paid search CPA is for most keywords.
In my view, both the mean and median are relevant to some extent, and a more actionable way to go about it is to compare the mean CPA with the median CPA. If they are significantly different, it means that there are some outliers in your data which you might want to address as priorities, whether they are too high and you want the cost down, or too low and you might be able to get more volume.
Standard Deviation, Or The Measurement Of Variability
The standard deviation is a measurement used for the amount of variability (or spread) in a data set. Essentially, it is the average distance from the mean, and it can be calculated as follows, although a simpler formula in Excel does the trick, too!
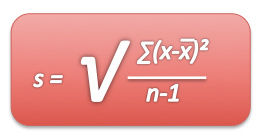
In this formula, x is the metric you are observing, x̅ is the mean; and n is the sample size. In Excel, you can use =STDEV(x values).
Applied to online marketing analytics, I can think of a couple of cases where you’d want to minimize the amount of spread as much as possible so you maximize overall performance:
Across multiple channels, if these x values are your CPAs per channel, you’d rather have all CPAs converge toward the mean, as opposed to having a wide range of CPAs across different channels. That is, you do not want to pay a CPA of, say, $25 through video ads while you can get a CPA of $15 in paid search — it just doesn’t make sense in terms of budget allocation and overall marketing mix optimization. Why not get more revenue volume from more efficient channels?
Within each individual channel, and for a similar reason, you do not want to pay a CPA of, say, $40 for some emailing campaigns targeting prospective clients while you could get a CPA of $20 targeting existing clients.You wouldn’t do that unless you are seeing long-term returns from those prospective clients’ campaigns (which is very likely but still needs to be measured), and/or there is no more room for growth for existing clients’ emailing campaigns. Another (perhaps more intuitive) example would be in paid search, where you’d want to lower the CPA for those costly keywords (mostly through quality score and bid optimization), and get more volume from those keywords with a low CPA (mostly through expanding your keyword list and increasing your visibility on less competitive or more efficient keywords).
In situations where you just observe and record the data (such as the ad spend per channel, or ad spend per keyword in paid search), a large standard deviation is not necessarily a bad thing; however, a small standard deviation is generally a good thing when it comes to your CPAs across or within channels in order to make sure you are getting a good amount of cheap conversions and a low amount of costly conversions.
In other terms, keeping an eye on the standard deviation is a good way to determine whether there is still a lot to optimize in your marketing program. More generally, you can calculate the standard deviation for your key performance indicators (KPI) and ask yourself whether the spread matters or not, and if it does, how you can address it and monitor it over time.
Standard Scores, Or How To Make The Data Relative
The standard score represents the number of standard deviations above or below the mean, without caring what the actual standard deviation or mean actually are. It is a slick way to put results in perspective without providing lots of details, which can get pretty handy when attempting to make sense of large data sets. Applied to online marketing analytics, standard scores can help evaluate performance for each individual value in the data.
The formula is as follows, where x is the metric you are observing, μ is the mean, and σ is the standard deviation.
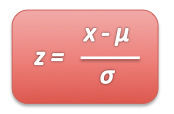
For example, you could use standard scores to quickly evaluate the cost for each individual keyword in paid search by comparison with all other keywords — while a cost of $60 might be hard to put in perspective, knowing that a keyword has a standard score of +2 tells you much more. This way, one can identify those keywords which need to be looked into first. In paid search, you could also use standard scores for those AdWords and Bing quality scores in order to compare the individual keywords’ relevance to the rest of the program.
Obviously, standard scores can be used in lots of different ways — those just mentioned are very basic. More advanced ways to leverage standard scores could include analyzing the relative performance by day-of-the-week (for instance, a Monday could be a +1 revenue-wise across all channels, that is, 1 standard deviation above the average daily revenue volume), or the relative performance by channel (such as paid search is a +2 efficiency-wise), and much more…
Also, in order to calculate those standard scores, just subtract each X value by the average X, then divide by the standard deviation. You could then end up with the below table for you keywords in paid search:

Percentiles, Or How To Bucket The Data
Another cool way to go about categorizing the data is to use percentiles, in other words buckets of data values with similar statistics, such as similar efficiency levels, similar revenue volume, similar quality score, etc..
More specifically, percentiles indicate relative standing as they help understand how individual data values are situated in your overall data set. Provided that the median is the center of the data, and by definition the 50th percentile, if you found that a paid search keyword belonged to the 90th percentile revenue-wise, it means that 90% of all other keywords generated less revenue.
Like standard scores, percentiles get very handy when bucketing your program by efficiency level and/or revenue volume, or prioritizing what needs to be optimized first, or just observing what is happening in your program in a more insightful way.
Unlike standard scores, there is no single definitive formula for calculating percentiles. Instead, you have to calculate each percentile separately. For example, if you want to find the 20th percentile, start by multiplying 20% by n (that is, the sample size) and then order your x values from smaller to larger. In this example, as there are 7,500 keywords in a report, we are bucketing those keywords in 10 buckets of 750 keywords, from smaller to larger CPA, and the 20th percentile would be those 20%*7,500=1,500 first keywords in the report.
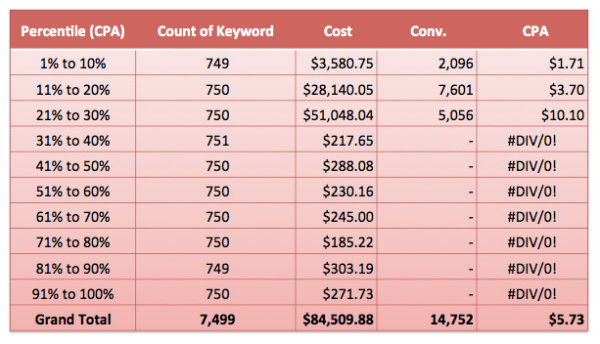
Across multiple channels, you could bucket all sorts of online marketing campaigns using percentiles based of each campaign’s efficiency.
Within each individual channel, you could identify top and/or poor performing campaigns/audiences/keywords based on any statistic that makes sense to you.
Conclusion
Hopefully, this post will bring light into new ways to analyze large amounts of online data. The key is to move away from presenting hundreds of numbers when attempting to analyze online marketing performance, as it can easily get overwhelming. Instead, relative standing statistics can make it quicker for analysts to put together, and easier for executives to comprehend and use for making decisions.
Comments