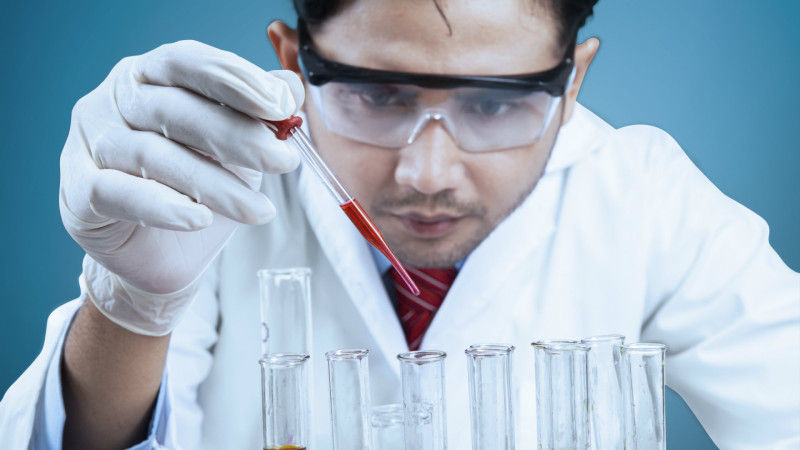
You’re a marketer … at least I’m assuming you are because you’re reading this. You take your data seriously. You pull daily, weekly, monthly, quarterly and annual reports. You analyze, forecast, and ultimately predict what will happen next based on what’s happened in the past and where you’re at today.
Analysts pull and analyze reports to understand why things happen. If our metrics were constants, then there would be no reason to pull so many reports because we’d be able to predict the outcomes. It’s the variation that keeps us on our toes when trying to understand what caused what and what we can do to capitalize on it.
Consider the table below detailing clicks coming from an ad placement:

My conversion rate is 2%. So it’s safe to conclude that people who click on the ad convert 2% of the time, right?
What if we ask the question differently: Did 2% of the visits yield conversions because they came from the ad? No. Why do we know that?
Because this report correlates clicks from the ad with a 2% conversion rate; it doesn’t validate it’s the ad which influenced the decision. In order to understand if the ad has any impact on user behaviors, we need to test and understand the causal impact of a user exposed to the ad.
But before we can test, we need to understand the difference between causation and correlation.
Causation Vs. Correlation
Observation: Billy was bitten on his arm by a mosquito. Three hours later, Billy developed a red, itchy dot on his arm.
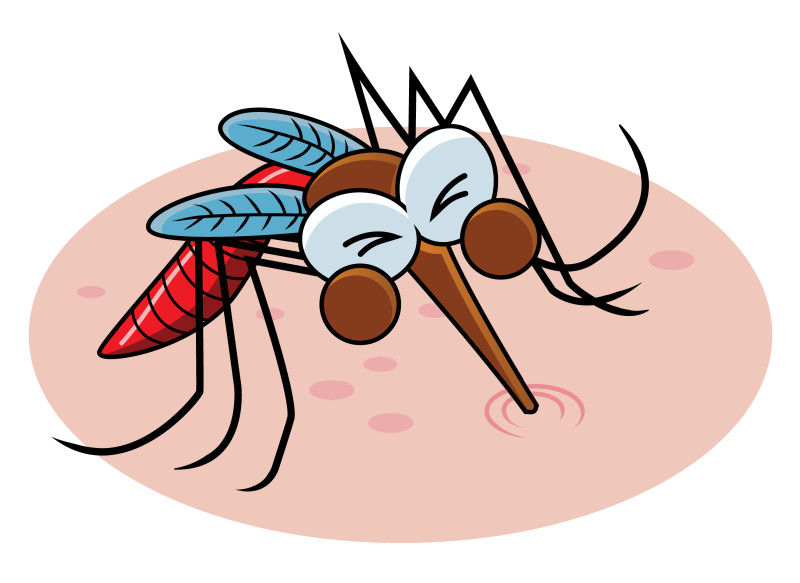
It’s determined after the fact that the itchy red dot developed as a result of the mosquito biting Billy’s arm. The mosquito bite caused the itchy red dot.
Observation: Every time Billy gets bitten by a mosquito, Jane gets bitten, too.
Here’s where we get into trouble. Is Jane getting bitten because Billy was bitten? There does seem to be a strong correlation between the two events, but even though they happen at the same time with regularity, it doesn’t mean one causes the other.
Now let’s put that into context.
Twenty percent of the time Acme Widget X is purchased from The Widget Store, the consumer subsequently purchases Acme Widget Y. Thirty percent of Widget Y purchasers have purchased Widget X in the previous three months.
Does that mean the purchase of Widget Y is caused by the purchase of Widget X? No, because not all purchases of Y are preceded by purchases of X.
But does it mean the purchase of Widget X is an indicator of increased likelihood to purchase Widget Y? For sure.
Most aggregated key performance indicators (KPIs) are also correlations, not causations:
Shoppers who visit the Acme Widget X product page have a 3% conversion rate.
Shoppers who click an ad for Acme Widget X have a 1.5% conversion rate.
The lifetime value (LTV) of a shopper with the initial purchase of an Acme Widget X is $250.
Based on the KPIs above, someone doesn’t have a 1.5% likelihood to purchase because they clicked on an ad; it just so happens that people who click this particular ad also convert with a frequency of 1/150 (1.5% of the time).
In order to measure the causal impact of an ad, we need to design an experiment to isolate a control group and measure the influence of the ad relative to not being exposed to the ad. In other words, we want to know the incremental value of a visit coming via the ad over and above a regular visit.
Consider the following experiment:

Does the ad cause the user to spend $100 2.5% of the time? No. Based on the control, a user is expected to spend $100 2% of the time. The ad increases the likelihood of conversion from 2% to 2.5%, meaning that the incremental value of the click coming from the ad is $0.50, not $2.50.
Any time we effectively predict, it’s because we’ve found some correlation between events that shows an increased likelihood of an outcome based on observed actions. Not surprisingly, many of the most commonly used machine-learning algorithms for prediction are based on pattern recognition, i.e., correlation.
Why is this important? Because you need to understand your KPIs in order to better inform your decisions. Testing takes time and costs money (whether it’s media or opportunity cost), but it’s the cheapest way to ensure your business decisions are more likely than not to be correct. Without testing, reports and observed KPIs are just correlations.
You can get by with correlations, but eventually it’s going to catch up with you. Get ahead of the game and start designing some proper experiments.
Commentaires