If you’re an online marketer that wants to improve conversions on your direct-response channel(s), this column will help you to create a multi-touch and multi-action predictive model for your conversion funnel that allows you to make better budget allocation and bid optimization-related decisions.
Start by focusing on two issues. First, not only should the end conversion numbers be tied back to all touch points in the funnel, but you also want to be able to track conversions across channels and use the adequate technology to do so.
Secondly, one should also use any meaningful upper-funnel metrics (i.e., pre-conversion or proxy metrics) in order to boost those upper-funnel touch points and ultimately drive more end conversion numbers.
Recent studies show that leveraging those upper-funnel metrics for low-volume keywords can help improve efficiency by significant amounts. Here are a couple of simple steps you can take to better leverage those upper-funnel metrics.
1. Analyze Historical Upper-Funnel Metrics Performance
This goes without saying: you need to have tracked those upper-funnel metrics for quite some time in order to analyze relevant data, especially if the conversion journey takes a long time. For example, say you’ve been tracking email sign-ups for a given e-commerce website for a year, and you’re interested in seeing whether email signups can help predict for the end revenue numbers.
The first report you want to dig into would be a report by keyword or product target in paid search, or a report by ad/audience for social and display advertising. You want both the end conversion metrics and any relevant upper-funnel metrics in here — such as email signups.
Now you want to plot the data in order to look at the relationship between end conversions and those upper-funnel metrics for each individual keyword/product target/ad.
If you use the total conversion numbers and upper-funnel numbers, you’ll get a lot of statistical noise because of some keywords or ads bringing much more traffic than others. To mitigate this issue, you should plot the conversion numbers per click against the upper-funnel numbers per click.
Another issue you might run into is those outliers, in which case you might want to trim the data if relevant. You’ll then be able to gauge how upper-funnel metrics might be associated with revenue for both low- and high-volume entities in the data. You should be able to visualize your conversion/click (revenue per click in this example) vs. upper-funnel metric/click as follows:
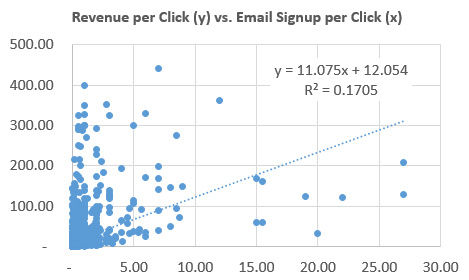
While the R2 (= determination coefficient) is fairly low in this case, you can still see some kind of trend. The slope being 11.07, we can say that 1 email signup is associated with roughly $11.07 of revenue. It is a rough estimate as variance is pretty massive here — but that’s a good starting point.
Also, one should be aware that we are talking about correlation, we cannot say that an email signup is the only cause for bringing some end revenue. But intuitively, it makes total sense that email signups help generate more revenue, so we’ll just keep those numbers moving forward.
Another way to go about it is to log transform those numbers in order to normalize the data and make it easier to interpret. As a result, we are now looking at log(Revenue per Click)=a+b*log(Email Signup per Click):
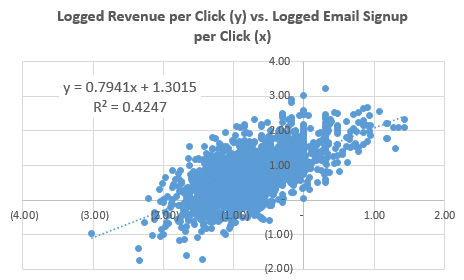
In this case, looking at the same data, we’re seeing a greater R2 which means we can be a little more confident with the model. From exponentiating both sides of the equation, we can say that one email signup is associated with roughly $3.67 of end revenue. While this approach spits out a better-looking model, it is unfortunately tough to use as it requires those extra steps of data transformation.
2. Identify Patterns From Upper-Funnel Metrics To Conversion
As stated above, correlation does not imply causation. A fine example of this is a set of keywords or ads which generated a significant amount of impressions, clicks and email sign-ups, but never generated any end conversions. For those guys — do we really want to predict any revenue at all? Maybe not, but intuitively those are doing better than other keywords/ads which do not generate any upper-funnel metrics at all.
Getting back to this email signup example, it seems reasonable to assume that email signups tend to bring between $3.67 and $11.07 of end revenue; however, we might want to make sure this type of logic is only applied to those keywords/ads which have generated both email signups and revenue in the past.
Another example I can think of where upper-funnel conversions do not necessarily predict for revenue is online dating free trials vs. paid subscriptions. Most keywords/ads tend to bring a lot of free trials, then paid subscriptions; but, a significant chunk only bring free trials. Generally speaking, you need to take this kind of phenomenon into account and identify clusters of keywords/product targets/ads/or audiences with similar characteristics, so you can build more accurate models for each individual cluster.
As a result, you might want to distinguish different channels and/or ad formats, brand vs. non-branded keywords in search, users who already like your page vs. do not yet in social, or users who already visited your site before vs. never, etc… The more clusters you end up with, the more accurate your models become; however, too many clusters can also dilute the data, so you need to find the right balance between clustering and collecting enough data by cluster.
3. Merge Those Multi-Touch & Multi-Action Models
The idea is that on one end, users go through multiple channels and devices before they actually convert, but they also do different things over the course of the conversion journey.
In a nutshell, online marketers need to comprehend what actions to expect prior to a conversion, and how those actions occur across multiple devices and channels over time. Once that story is well put together, one should be able to better predict the end revenue numbers based on early upper-funnel metrics.
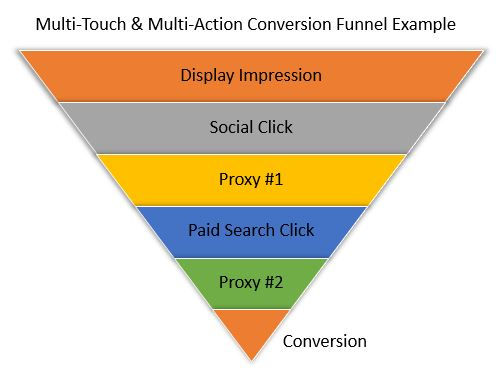
Conclusion
It is going to take a couple of tries before you end up with a ‘good enough’ multi-touch and multi-action predictive model — you want to see how well your models predict, then adjust them over time.
Some technologies such as the one we use at my company do just that: predict based on both end revenue and upper-funnel metrics, verify predictions, and fine-tune models accordingly. I feel this is the way to go in a world where data have become highly complex, and predictive models are being challenged every single day.
Comments