The discipline of marketing science arose from marketers’ number one need: to know what advertising efforts work. What are the forces that drive sales up or down, and by how much?
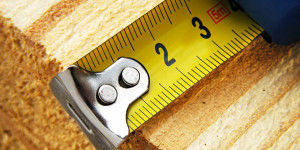
To answer these questions, marketers need data. In yesterday’s world of mass media broadcast advertising, aggregated data was all that marketers had to make data-driven decisions.
It was enough for marketing scientists to develop sophisticated econometrics models to fuel an entire discipline known as marketing or media mix modeling (MMM).
Marketing (Or Media) Mix Modeling (MMM)
These models use historical information to analyze the incremental impact of various marketing efforts on sales. They are complicated statistical models aimed at creating a regression-based relationship between the marketing activities and sales results, analyzing the contribution of each piece of the puzzle as a percentage of the total results to determine the effect that channel had.
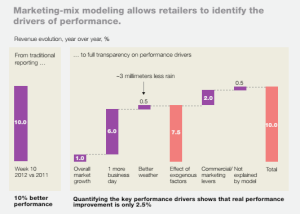
Illustration of MMM from McKinsey report (PDF) Click to enlarge.
MMM models, which were originally developed in the late 1980s, are still used today — especially by big spenders like consumer packaged goods (CPG) marketers — to deliver powerful high-level insights to the forces that drive sales.
Some of these drivers are media channels that marketers can influence, such as TV advertising; others are completely external and independent, such as weather.
MMM insights are typically used to do strategic scenario planning, set annual budgets and optimize the marketing mix at a high level.
While these are incredibly useful pieces of information, they leave marketing departments and agencies asking questions like, “Okay, we know we need to spend $500,000 more on digital. But how, when, where and to whom? Display? Retargeting? And if so, which network and creative? If search, which keywords?”
MMM struggles to provide sub-channel, tactical-level insights due to the intrinsic limitations of using aggregated data and econometric models. Some of the most critical limitations include:
The risk of predictors being highly correlated with each other, which makes it difficult to measure media at the tactical level
The crude measurement of dynamic and fast-changing digital and direct marketing channels
The inability to measure the performance of new products and campaigns, which lack historical data
Multi-Touch Attribution (MTA)
Fortunately, today’s technology now allows marketers to measure advertising performance with incredible accuracy and granularity thanks to a new technique: multi-touch attribution (MTA).
MTA, the foundation for the technology at my company Convertro, was born out of advanced web analytics technology. Initially leveraging browser cookies, online businesses realized that they could gather incredibly precise and granular data on customer acquisition and retention. Technology expanded further to cover issues like cookie deletion, cross-browser navigation and cross-device behavior.
Access to user-level data allowed data scientists to develop predictive models with actionable outputs such as “send an email to user x, y and z to increase conversions by 2 percent” or “lower bid on this specific keyword by 30 percent to get a 3 percent lift in return on investment (ROI).”
In other words, where MMM modeled aggregated data to predict the effect of advertising on consumers, MTA observes real consumer behavior and (with some major help from big data processing) determines exactly what matters.
MTA takes what could be a digital-era challenge – the cross-channel, cross-touchpoint relationships between brands and consumers – and leverages it as an advantage.
A consumer might view an ad on Facebook via a smartphone, then later log into her account from her home PC to do some additional browsing, and then make a purchase a few days later on her tablet. A robust MTA solution will not only recognize those actions as all belonging to the same consumer, but will also quickly indicate which interactions played a role in any eventual purchase.
Bottom-Up Vs. Top-Down Solutions
In a world where consumers spend more time online than they do watching TV, it’s easy to see why attribution is gaining so much attention. While aggregated data and channel-level recommendations were sufficient yesterday, they struggle to keep up with today’s micro-targeting and demand for speed and granular recommendations.
Although it’s easy to aggregate granular data into higher-level analysis (the so-called “bottom-up” approach, typical of MTA solutions), it’s simply impossible to extract granular details from already-aggregated data, and that’s why MMM feels limited in its broad-level “top-down” approach.
Another advantage of “bottom-up” approaches is the ability to analyze data almost in real time, and to use software to deliver scalable channel and sub-channel performance analysis that used to take months. By contrast, MMM requires an expensive custom-made model for every business.
How is the market reacting to these changes? MMM providers are quick to point out the limitations of MTA, including its general inability to measure any channel that does not allow for user-level data collections, such as print or out-of-home, and to account for externalities.
These providers highlight the sophistication of their models, capable of accounting for many custom variables such as weather and competition. Nevertheless, they are under extreme market pressure to measure digital with the speed, granularity and accuracy demanded by the advertising industry.
Most MMM providers know they need MTA data to refine the digital slices of their media mix pie, and in fact, many quickly came out with a parallel MTA product by buying data or partnering with MTA technologies (MTA analysis requires robust big data platforms and specialized engineering expertise that are difficult to build in house, even for large brands and agencies).
What’s Wrong With Using Both?
For lack of a better solution, many brands are now hiring both MMM and MTA providers. This is far from efficient. Indeed, this is only one of many instances where marketers are presented contradicting truths by vendors and consultants.
After all, different display networks claim credit for the same conversions; Google has been able to sell products that contradict each other for years now. But times are quickly changing. The most obvious market force is the unstoppable rise of online commerce (and therefore, advertising) at the expense of physical retail.
Technology is pushing toward digital advertising, too: not only have smartphones completely changed how consumers shop making MMM models slow and obsolete; but, it’s clear that consumer-level data will soon be available for channels that have traditionally been measured only with aggregated data such as direct mail and TV. If in five years, every household will have set-top boxes turned on, TV could be attributed from the bottom-up with the same speed and accuracy of digital channels.
At the same time, the market is pressuring MTA providers to deliver more sophisticated models, accounting for brand awareness, seasonality and offline channels, to reach the “holy grail” of marketing science: measuring the holistic impact of advertising on sales.
Will MMM models become increasingly irrelevant as the market shifts and the technology develops to make MTA measurement possible across all campaigns? In the near future, I believe incorporating both models will only serve to confuse marketers.
Who Wins The Rush For Innovation?
In the end, both approaches want to measure and predict the incremental revenues generated by introducing one or more channels, or shifting spend inside or across media, as well as give marketers the confidence to justify ad spending and inform future media planning.
With both options available, marketers might want to still rely on MMM for annual budgeting and optimization of channels where consumer-level data is not available, such as print and out-of-home, but embrace a more accurate MTA solution for all things digital and TV.
It’s an exciting rush for innovation, and the winner will be, ultimately, the one that masters the best of both worlds. However, the recent acquisitions in the multi-touch attribution space, inclusive of Convertro by AOL and Adometry by Google, are signaling that attribution might be the natural evolution of marketing mix modeling, particularly as better consumer-level, real-time data become available across online and offline channels.
Comments