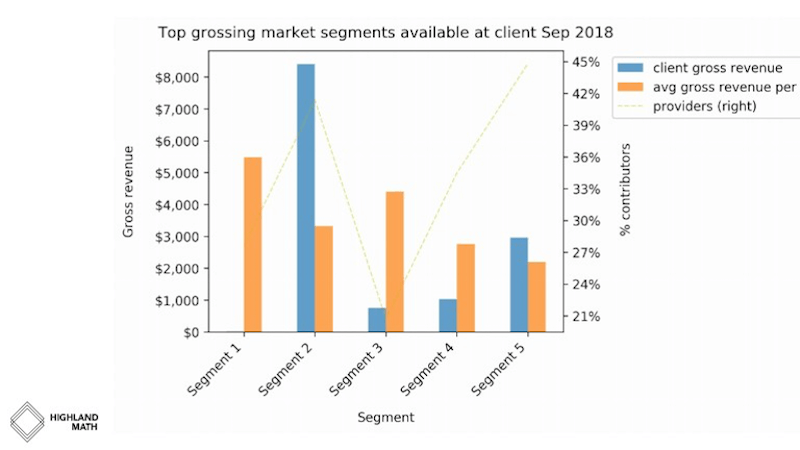
Marketers buy and use audience data every day, but how segments are priced or labeled by data providers is one of the mysteries of the data market.
That’s where startup Highland Math comes in. Based in Arlington, Massachusetts, it was founded last summer to create an unusual entity: a co-op of data providers that only sells intelligence about data transactions, not data itself.
The co-op model. CEO and co-founder Dan Scudder, who also helped start and was General Manager of identity resolution provider LiveRamp, noted differences between his company and various other data coops. They offer a data marketplace or provide intelligence about a given sphere, he said in an interview, whereas Highland Math offers an intelligence layer about all kinds of data transactions.
ComScore, he pointed out, has a similar model, but it is focused on measurement and market share for media placement. A classic data marketplace like Oracle Data Cloud, he said, can provide insights, but only about transactions involving Oracle Data Cloud.
Bombora manages a cooperative of publishers that collects intent data on site visitors, not transactional info on data sales. Affiliate marketing network Skimlinks maintains a data co-op that shares targeting data on customers and visitors among participating publishers.
Sharing transactional data. By contrast, Highland Math is designed to provide aggregated intelligence to buyers and sellers of data, such as pricing, popular segments, names for similar data segments, advertiser buying trends and so on, tracked through transactional data. The participating data providers, which currently number 40, share their transactional data in exchange for receiving insights.
Participating providers, which are primarily US-based but whose products often offer global data, include providers of offline data, behavioral data, location data, B2B data and college-related data, such as Epsilon, ShareThis, 33Across and Alliant.
Scudder gave the hypothetical example of Data Provider A, which sells an audience targeting data segment of consumers who have recently moved, for use through Google’s ad platform by an advertiser like Home Depot.
Data Provider A will share with Highland Math when the sale was made, the price, the segment name (such as New Movers), the advertiser, and so on. The shared info is almost entirely from the sell side, Scudder said, not from the capture side about how the data was obtained.
Typical use cases. Members of the coop buy the insights about sales through a subscription that is based on the volume of insights. Categories and other reporting is normalized across platforms so that, for instance, data about all recently-moved consumers has the same label.
The co-op data covers transactional activities on about 70 platforms, including demand-side platforms, data management platforms, data marketplaces, analytics platforms and others.
For a typical use case of these insights, Scudder recalled an unnamed client that was “underperforming when targeting by gender.” Highland Math’s insights on transactions, he said, “helped them understand [how their] pricing worked,” discovering that their datasets were overpriced compared to competitors’. By lowering their price, he said, they doubled their sales.
Another client company, he said, had mislabeled a data segment about people who owned homes. “We saw that segment was popular,” Scudder said. When called Homeowner Data, it sold better.
Why you should care. Scudder said that, in the second half of this year, his company will move beyond data sellers and begin to provide insights directly to advertisers, who often have to choose among thousands of audience segments to target their ads. Those segments might include, for instance, three dozen segments that are described as consumers who have recently moved, and Highland Math will provide guidance on which one to choose for best performance.
In the meantime, data sellers who can use market intelligence to target their pricing, segment labels and other variables at what marketers want — and are willing to pay for — can help make the black box of data selling more efficient.
This story first appeared on MarTech Today. For more on marketing technology, click here.
댓글