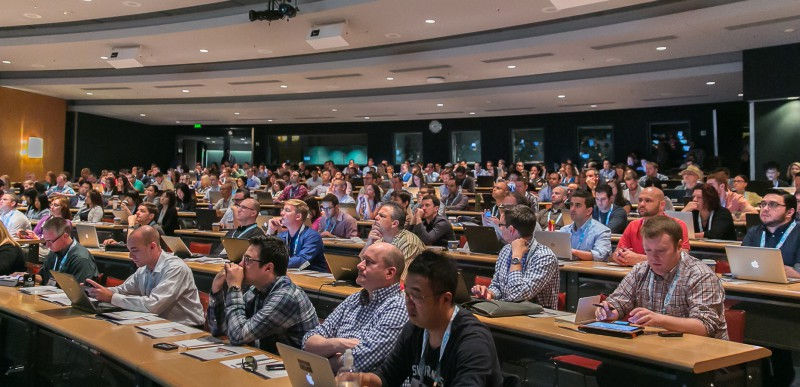
At SMX Advanced in Seattle this month, Lin Huang, senior data scientist at Microsoft, presented an intriguing experiment the company has been running. It involves analysis of social media to provide actionable product and brand insights.
The goal of the method of analysis it has developed is to help brands better target ad spend and ad content to their core strengths as seen by their customers and fans.
The following is a summary of Lin’s presentation.
Brand Perceptions From Social Media
It is rarely the case that the complete story of what fuels a purchase decision can be traced by a series of search queries alone.
Opinions expressed about a brand or its products by people in the social circles of a buyer can play a major role in the buying decision. What is too often overlooked is how valuable those same opinions might be for identifying brand/product strengths.
Social media is not really a competitor to search engine marketing (SEM). In fact, social media can actually be a rich source for advertising content in at least three ways.
Buzz generator: Advertisers can note what gets talked about most and optimize on related keywords.
Targetable audience: Analysis of characteristics of people who talk about your brand on social can provide clues for targeting you may have missed.
Brand equity extractor: The main value that the Microsoft methodology extracts, explained below.
In summary, social signals can help brand owners understand what really resonates about their brand and products, as well as how they are judged by real people compared with competitors.
How Social Networks Feed Brand Perceptions
In the image above, we see a typical product page from an e-commerce site, with the ability to filter products by certain features, in this case screen size, hard drive size, and weight. But look at the tweets on the right. These people are revealing their expectations when purchasing a laptop.
Notice that Dennis doesn’t care about the size of the screen; he wants a certain quality of display. Other tweeters are looking for other features, none of which are in the filters on the e-commerce page.
Takeaway: What consumers think is important about your brand and products might often be different from what you think. Use social listening to discover those consumer insights, and then adjust your site and product offerings accordingly.
Mining Tweets To Build Advertising Strategy
Brands often use conduit studies based on features. But what if there are features that are important to potential customers that are not in the set you are studying?
In 2011 Thomas Lee and Eric Bradlow published a study in the Journal of Marketing Research showing how to use data mined from customer reviews to better structure marketing campaigns. That paper inspired Huang and his team at Microsoft to study how similar data taken from consumer tweets could be used to enhance the effectiveness of online advertising campaigns.
The Microsoft study started with text mining of tweets and translated them into actual feature sets. Potentially, brands could gain from this data:
Better understanding of new feature sets.
Improved knowledge of how their offerings are judged vs. competitors.
End result: better targeted SEM campaigns.
Case Study: Major Wireless Carrier
Huang and his team started by generating a word cloud from relevant tweets.
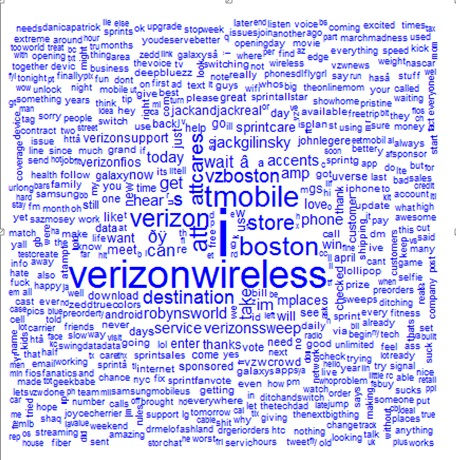
They then used sophisticated techniques to extract meaningful phrases that showed what people were really talking about.
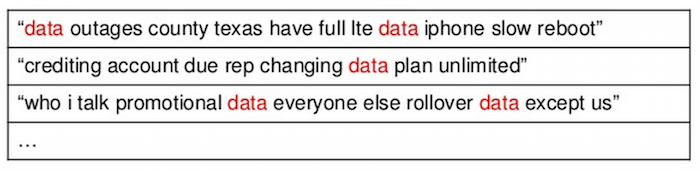
Using cluster analysis, they grouped the filtered tweets into color-coded clusters related to a particular feature.
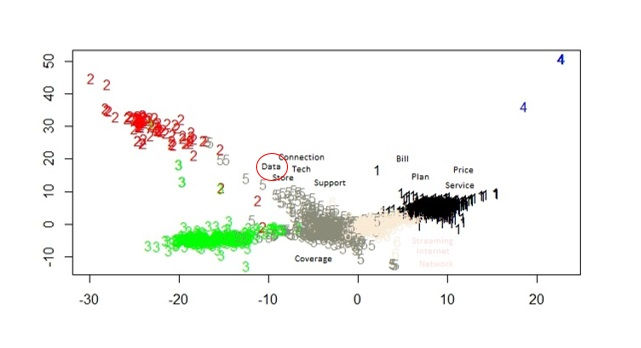
That data was then transformed into a two-dimensional graph.
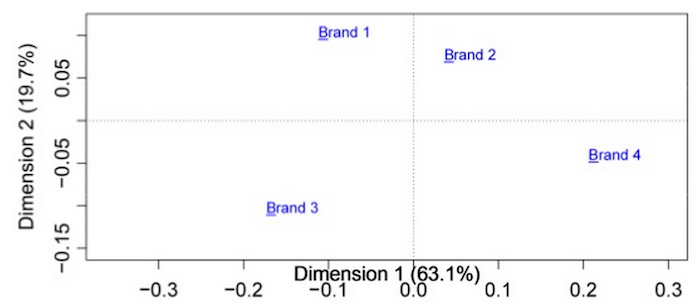
Dimension 1 graphs the elements every consumer thinks about for wireless services (price, options). Dimension 2 charts the more hedonic aspects, such as quality of customer service. The graph gives a picture for four brands.
The Microsoft team’s insight from this chart was how it could be used to differentiate each brand and therefore help those brands better focus their ad bidding.
Notice each of the four brands happens to occupy one quadrant of the graph, which means there is great difference in perception of these brands by consumers. Consumers don’t really see them as close competitors, so imitation and price wars won’t work.
Instead, these brands should emphasize the features where consumers see them as superior to their competitors. Practically, that leads to knowing what keywords and ad copy to emphasize in bidding.
Further analysis of the study generated several more insights and discussions among the Microsoft team members, including:
Who generated the social signals matters? How do we weigh them?
How could this data be packaged with other information to better inform ad buying?
How should they resolve conflicts between this data and those other information sources?
This data could be valuable for more than just SEM advertisers.
Here is Lin Huang’s slide deck from his presentation at SMX Advanced:
About Lin Huang
Lin is a seasoned marketing researcher, mathematical psychologist and statistician with 13 years of experience in deciphering consumer behaviors. In Microsoft, he leads Bing Ads Advertiser Experimentation & Program Measurement Initiatives, and has pioneered exciting breakthroughs in conversion attribution, social influencing and many complex advertiser experiment designs.
Before joining Bing Ads, Lin did macroeconomic research at the central bank of China and taught marketing class at the University of Michigan, Ross School of Business. He regularly trains internal Microsoft analysts on a range of SEM and technical topics. Lin’s research has appeared in leading academic publications including Advances in Consumer Research and International Symposium on Forecasting Proceedings. Contact Lin.Huang@microsoft.com
Comments