Correlation Or Coincidence: The Race For Causation In 2016
- Fahad H
- Feb 9, 2016
- 5 min read
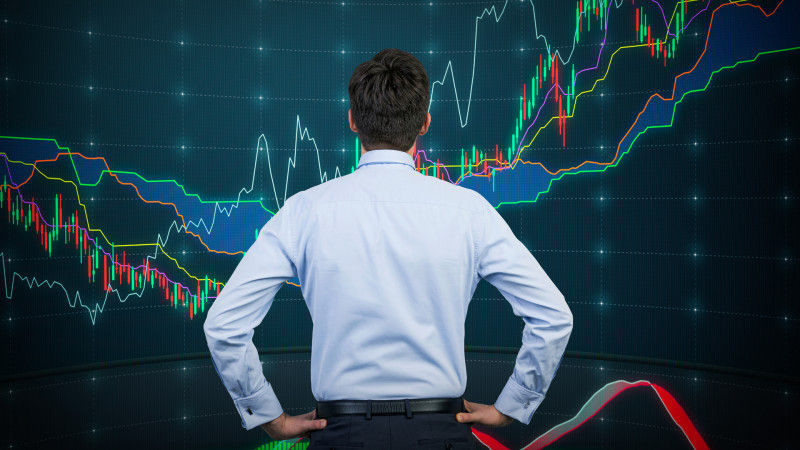
For marketers, 2015 was the year we figured out that buzz and sentiment were as likely to lead us astray as to offer insights that move the needle.
That means the race is on in 2016 for marketing analytics professionals — both in-house and vendors — to figure out how to find data that intelligently correlate to revenues (or at least, to KPIs that are smart approximations of revenue, such as units shipped, subscribers and so on).
But beware. In any race, there are shortcuts. And some shortcuts can lead to disaster, not discovery. That’s exactly what’s starting to happen in the world of marketing analytics in 2016.
In many ways, marketing analytics vendors are starting to sound the same. Each one promises to help marketers impact revenue and prove their value to their respective C-suites.
And with stunning visualizations and alluring dashboards, it’s easy to create the appearance of “signal” versus “noise” the moment social media measurement graphics begin to take the same general shape as revenue charts. After all, the human brain is genetically programmed to assign meaning to shapes and draw conclusions from special similarities.
But caveat emptor: Simply layering a financial graph on top of social trends doesn’t reveal why financial outcomes occurred. In fact, it’s as likely to mislead marketers as to help them.
There’s a lot of hard, backbreaking data cleaning and a mind-boggling volume of testing and re-testing that go into discovering meaningful correlations. Marketers who want to avoid following statistical fireflies into the forest should learn how to discern coincidence from correlation.
Here are a few pointers for the discerning marketer:
Correlation Isn’t Causation, But Sometimes It’s Hard To Tell The Difference
Everyone knows correlation doesn’t always indicate causation. But it’s not always easy to apply this principle in real life.
In November and December, for example, social chatter surely includes peak references to Santa and a high point in sales for many companies. If these companies were to overlay social buzz onto their financial data, some of them would see what looks like a series of correlations between Santa and sales.
Does this mean Kris Kringle is driving revenue? Probably not. Someone might tweet, “I’ve been good this year — think Santa will bring me a Ferrari?” But that doesn’t mean this person (or anyone buying him gifts) is a likely Ferrari customer.
Correlations aren’t useful unless they’re thoroughly validated to remove noise. Some short-term correlations may appear causal, when they’re actually just random alignments of unrelated variables.
Validated correlations must undergo significant vetting, such as using them to generate statistical models that are repeatedly tested against past data.
Takeaway: Apophenia, or the human tendency to find connections and meaning in unrelated events, is real. Similarly, Confirmation Bias, or the tendency to focus on data that confirm an existing belief, is another distortion in human perception. Simplistic correlations encourage us to see what we want to see. Correlations built from sophisticated data science challenge our assumptions and invite us to explore what’s really happening.
Unstructured Data: Messy And Need To Be Cleaned
Causal factors are relatively easy to find when you’re dealing with only two or three variables — but with unstructured datasets, the variables are endless. Most of the data are useless, which means the dataset needs to be cleaned before any meaningful correlations can be discerned.
The raw dataset from any social media source includes song lyrics, millions of retweets mindlessly promoted by legions of bots, marketing messages, spam and more. A cacophony of garbage drowns out real consumers sharing their unaided, organic thoughts. In our own work, our company has found that more than 80 percent of what shows up in social media listening offers no value.
To build trust in correlations between consumer data and revenue, marketers need to understand how the data are cleaned and whose statements they ultimately represent.
Remember, the goal is to use consumer data to understand how marketing strategies are impacting revenue. If your data are polluted, your insights won’t reflect your actual customers, and that big bet you’re about to make will become a big mess.
Takeaway: Of all the data you could be looking at to help you find what’s driving your business, on average, only 20 percent actually matters. The trick is to work with the 20 percent that matters and weed out the 80 percent that doesn’t. And that starts with taking out the trash. Data need to be cleaned. If you aren’t taking out the trash, your correlations will be made of junk.
Strong Correlations Shouldn’t Dictate Strategy; They Should Encourage Exploration
Even the strongest, most thoroughly validated correlations can’t be magically automated into impactful strategies. Good correlations aren’t a replacement for human curiosity, creativity and intuition. They’re guides.
Any vendor that claims to serve up visualizations of “causation” is peddling snake oil.
Let’s face it: Causation is eternally elusive, and, for now at least, defines statistical certainty. Some leap of faith will always be required for a marketer to decide to take action. (And thankfully, that’s what the gorgeous organic algorithm known as human intuition is for.)
Even in controlled environments, we’re rarely able to determine beyond doubt that one thing caused another — that there couldn’t be some additional variable we didn’t think of and couldn’t detect.
In other words, strong correlations aren’t a sign of what’s definitely working. They’re statistical anomalies that, after thorough validation, trigger the human curiosity algorithm in some brilliant human being and demand exploration. They need to be interpreted and explained in context by people who understand their business, their consumers and their markets.
That’s why you shouldn’t look at a predictive model, which only tells you what’s likely to happen, or a prescriptive model, which only tells you what to do. The preferred method is an explanatory analytics model, which lets you explore possible explanations for why certain business outcomes are occurring — and the best ways to improve those outcomes.
Takeaway: Technology can calculate the most likely relationships among scattered variables. It can weed out whatever is definitely not contributing to a given business outcome, and it can serve up intuitive visualizations of the most likely suspects. But ultimately, it’s up to an experienced professional to pick the real causal culprit out of the lineup, decide to take action and form a brilliantly creative strategy based on those insights.
So when kicking the tires on any given analytics solution that claims to offer actionable insights, here are a few questions to ask:
How does my analytics solution guard against confusing coincidence with correlation?
How do we find the 20 percent of data that matters to help reveal useful insights?
How does my analytics solution validate correlations, once it’s found them?
What role does my own curiosity and intuition play in this platform?
How can I use strong correlations to ask deeper, better questions?
What’s the relationship between my analytics and my creative marketing processes?
Yorumlar