There are few issues more important than customer retention when running software-as-a-service businesses. It’s no good acquiring customers for $10, if they only stick around for a month or two.
Measuring retention
Retention can be measured qualitatively by talking and checking in with your customers regularly so you’ll know what their main questions and issues are, and when they occur. Retention can also be quantified, giving you hard data on how much money you’re losing. Typically this is done using a cohort analysis.
Download The Growth Handbook
Authoritative, actionable strategies on how to grow your business.
What is cohort analysis?
A cohort analysis is a technique borrowed from medicine to see how variables change over in different groups with different starting conditions. Retention is a simplified one, where the starting condition is usually the time of sign up and the variable is simply activity. You see them visualized like this.
If you’re looking to add some functionality, the most useful ones are allowing highlights of drops, and letting the user switch between relative and absolute figures. Like so:
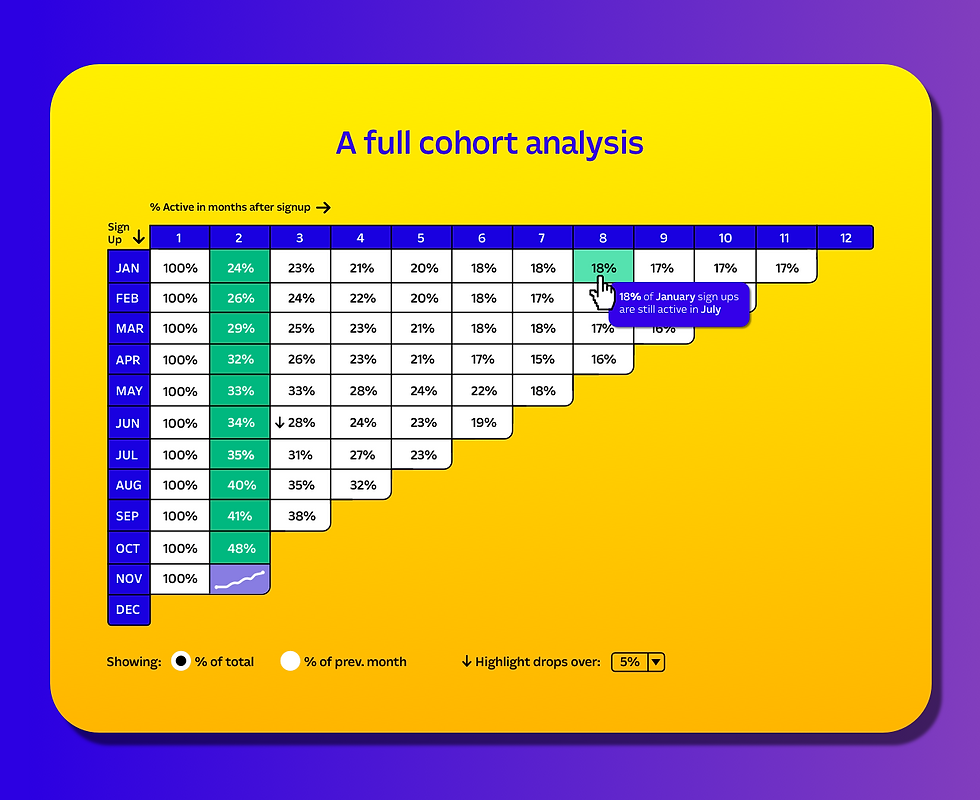
When I started designing cohorts for applications, something always bugged me about the visualization. When you’re looking at a cohort you have a few different questions:
How is our retention rate overall?
Where do we lose customers?
Is the rate at which we lose customers getting better or worse?
Whilst the above grid of squares can be useful, and augmenting them with sparklines helps, there are still comparisons that are difficult to make.
Why visualize retention data?
The purpose of a data visualization is to highlight what can’t be seen otherwise. Just spitting out data in squares is really just a fancier version of Excel. In some cases it’s more important that the viewer sees the comparison, not the data. In this case, if our question is simply “Are more customers sticking around for a second month?” then we should focus on that solely. Like so:
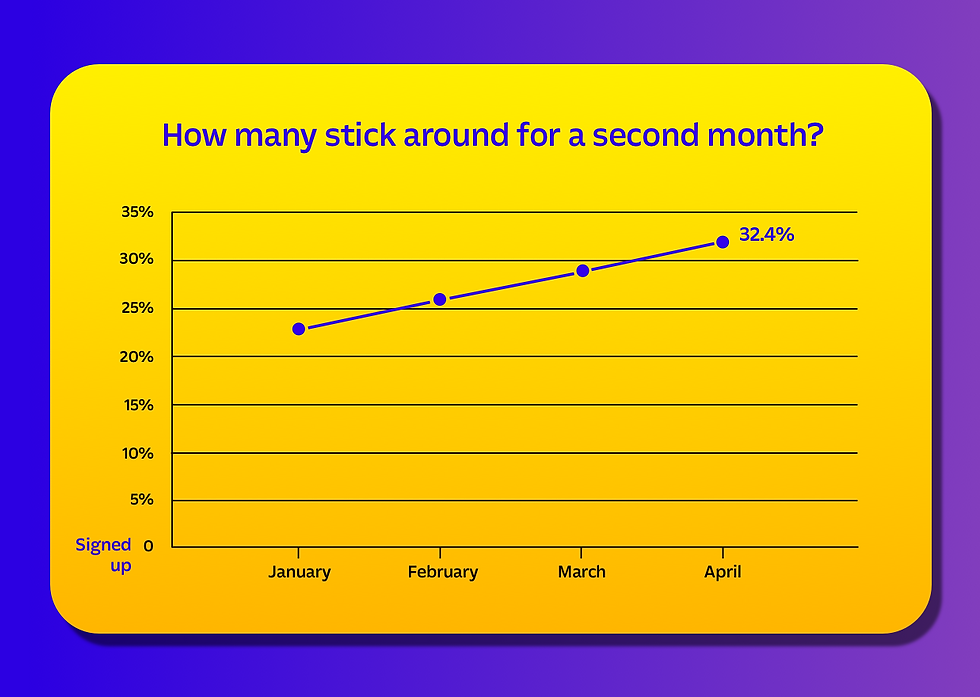
This chart makes it very clear that we’re increasing our retention rate. Whatever changes we’re making to our onboarding seem to be working. However, this chart only looks at one sliver of the data. We could be fooling ourselves.
Gamification, hacks and promotions all have great short term effects but it’s lasting value we’re after. Adding a marketing email on day 31 might trigger another wave of logins, but do these guys really come back, or is it just superficial? How can we see that? Well we could produce one of these charts for every retention rate (1 month, 2 month, 3 month, etc). That would be cumbersome, but would work. We could also try something different.
Using cycle plots to plot retention
Cycle plots are a technique I first learned about from a paper(pdf) by Naomi Robbins (found via Stephen Few), and they work by grouping the data points together showing both micro and macro trends.
Remember our retention graph above? Let’s plot our retention rates for months two, three, four and five all on the same graph.
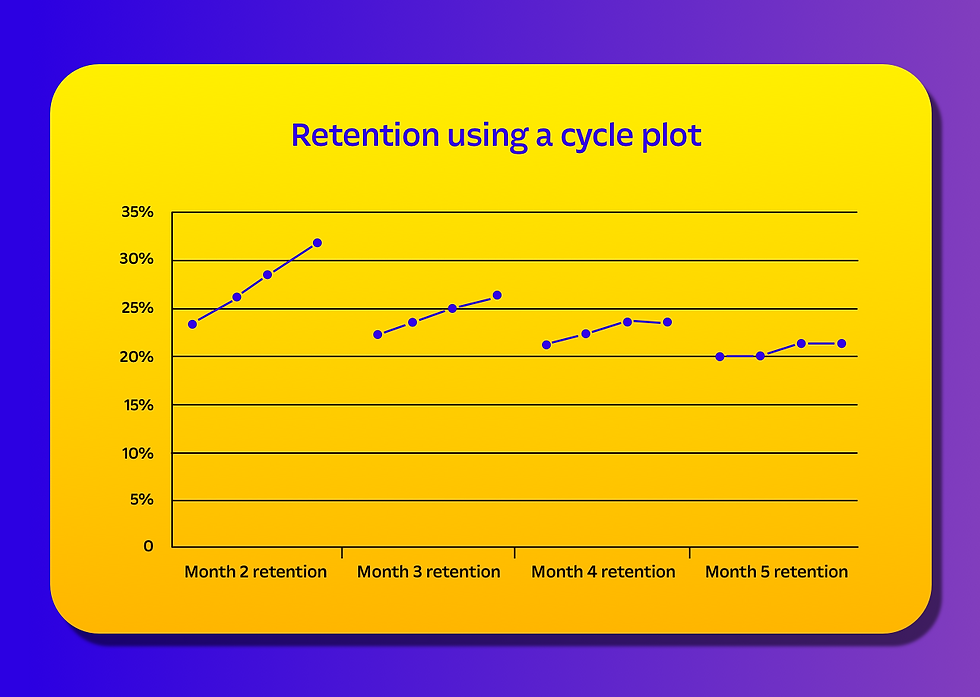
Note how we can now see every data point, the retention trend for each month and by taking the clusters as single data points we can see the overall trend of retention.
We can take this further and colour code the points to make more comparisons easier. It is worth pointing out that these charts aren’t immediately understandable to someone with zero knowledge of cohorts, nor is that the goal. The goal is to empower someone doing cohort analysis. We can add to this by making the charts interactive, disclosing extra data on hover and other such additions.
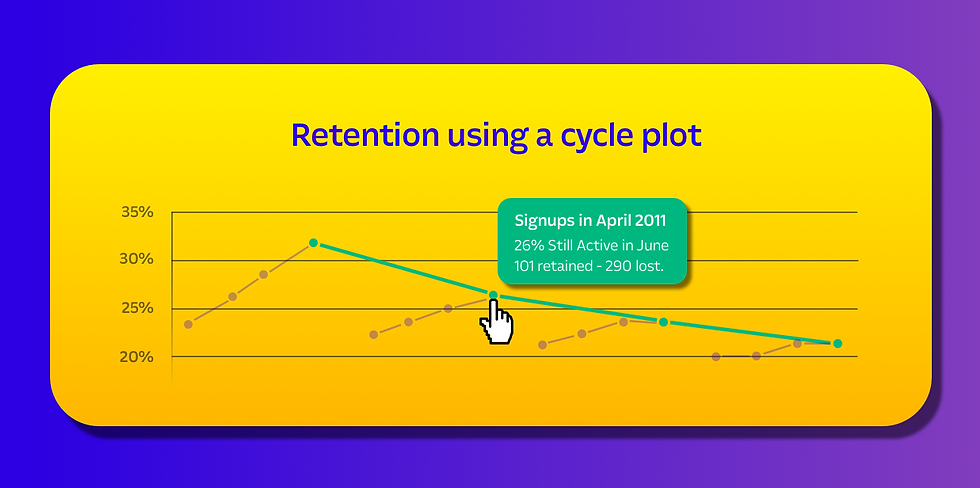
Cohorts are useful for visualizing where customers are lost. If the results of a cohort analysis shock you, then you’re not in regular enough contact with your customers. Solve that problem first. There’s no use knowing exactly what’s happening if you don’t know why.
Comments