The Digital Marketing Analysis Tree: Understanding Root Causes
- Fahad H
- Sep 26, 2013
- 3 min read
I recently read an excellent article in the Harvard Business Review about how data-driven individuals think and act. Some of the key traits described in the article apply not only to researchers, but to anyone involved in analysis and decision making.
According to the author, data-driven individuals:
Make decisions at the lowest possible level
Bring as much diverse data to any situation as they possibly can
How do these traits translate for the digital marketer, and how we should think about performance management of campaigns?
The Data-Driven Marketer
The traits, when applied to marketing, suggest a data-driven marketer tasked with performance assessment should:
Try to narrow down the scope of impact as much as possible — even to the individual target, keyword, placement, or creative level
Not only look at top-level KPIs such as revenue or ROI, but dig deeper into more granular metrics in order to assess where exactly the performance improvement or decline is coming from
Conducting such an analysis often takes some time, so there will be cases — particularly for young campaigns — where rapid turnaround has the potential to achieve greater impact than in-depth investigation.
However, as campaigns mature and settle into a steady state, the amount of “low-hanging fruit” gradually decreases. This necessitates a shift of focus from speed to accuracy, which means that it becomes increasingly important to make solid, data-driven decisions.
Root Cause Analysis For Digital Marketing
Broadly classified, the need for performance analysis in digital marketing occurs under one of two scenarios.
One involves experimentation, where hypotheses are formed and tested against control groups. In this case, interpreting results is relatively straightforward, since almost all of the work was completed during the planning phase.
The other scenario — appearing far more often in reality — is when performance changes occur under uncontrolled situations, and there are a myriad of different factors that could have impacted the change.
My preferred approach to this type of investigation falls under a method known as Root Cause Analysis (RCA), which is used across a number of industries and business functions to determine causal factors of events and to identify appropriate response actions.
The Digital Marketing Analysis Tree
One way of conducting RCA analysis involves a causal factor tree, linking higher-order outcomes to lower-order causes. For marketing, this can be represented as a hierarchy of KPIs and metrics. One example of such a tree for paid search is shown below.
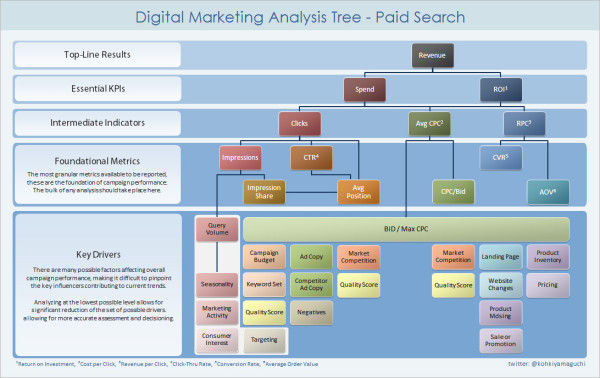
Click for larger view
The objective behind this approach is to break down higher-level KPIs into more manageable, foundational metrics, each of which is only impacted by several factors at a time. This allows us to better assess which factor(s) contributed to a performance impact.
This approach is applicable not only to paid search, but for any digital marketing channel with a hierarchy of metrics. For instance, metrics such as impression frequency come into play for programmatic display and social, whereas other metrics like position fall off.
For unit-based KPIs such as orders, the tree will look very similar except with CPA (cost-per-action) replacing ROI, breaking down into CPC (cost-per-click) and conversion rate.
The Importance Of Granular Analysis
In a live campaign, external factors such as market competition, seasonality, and inventory changes are constantly affecting performance, creating results that can be misleading when observed on a higher level.
While controlled experiments provide a definitive answer to any single question, there is often not enough time or budget to design and set up experiments for all initiatives executed against, and they cannot help with spontaneous performance changes.
RCA represents a data-driven approach for breaking down the chaotic digital marketing environment and identifying the root cause(s) behind performance changes. Over a long term, this leads to more accurate learnings and better optimization.
Comentarios