Statistical Tricks You Need That Prove You Should Read This Column Every Month
- Fahad H
- Jul 30, 2013
- 7 min read
The folks at Search Engine Land spend a lot of time trying to divine the Google search algorithm from Matt Cutts’ words like reading tea leaves in the bottom of a cup. All the while, several of my brilliant colleagues and I from the Search & Conversion column have been talking about what to do with the traffic the Almighty G bestows upon our sites.
On Search Engine Land, we were somewhat limited to topics directly or indirectly related to search marketing. Now, we can spread our wings here at Marketing Land. Represent!
With a completely new audience, I’d like to entice you to read this column regularly by subscribing to my Twitter feed. I’m going to do this using the same analysis tools we use when trying to understand what our analytics data are telling us. Then, I’m going to show you how to present the data pointing out salient points. There may be some spin in this process.
All of this can be done in Excel, so fire up your spreadsheets.
The Data Set
The statistics surrounding my columns on Search Engine Land are “time series” like most of our analytics data. The horizontal axis, or “x” axis is measured in days, weeks, months or dates.
The data about the columns have 46 data points over 3.62 years, which is manageable as an example. That’s 46 columns, one every four weeks. That comes to about 46,000 words in total.
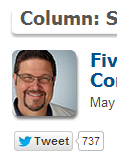
The fine folks at Search Engine Land have given me their pageview data on my columns. I was also able to collect some data by looking at the number of tweets registered by the twitter badge on each page, and by counting the comments on each column.
Conclusion number one: That I’ve written for “almost four years” demonstrates the kind of credibility and authority that will get you to tune in. “Brian Massey. Serving the marketing public since 2010.”
Validating The Data
The first thing we need to do is get a confidence level in our data. The first way to do that is to “eyeball” it. I like to do a quick line graph of the data.
Here’s a graph that includes data provided by Search Engine Land.

The reported visits to my columns look suspicious.
Notice that there is a significant change in July of 2012. Did I “get on the map” at that time? Is the data before that not valid?
The folks at Marketing Experiments identify the kinds of errors that affect your data. Two that we want to consider here are the “History Effect,” which identifies natural changes over time, and the “Instrumentation Effect,” which identifies errors in your measurement process or tools.
If I suddenly became popular in July of 2012, that would be a history effect. If Search Engine Land changed the way it collected data in July of 2012, that would be an instrumentation effect.
Without knowing which is true, we need to get a second opinion.
When In Doubt, Find Related Data
There are two other sets of data that can be brought to bear on this analysis: Number of tweets reported by the Twitter badge on the page and the number of comments. The folks at Search Engine Land have noticed a relationship between Tweets (as reported by the Tweet badge on each page) and unique visitors. For every 100 Tweets there will be about 1,000 unique visits.
If we use that assumption and graph the visits predicted by tweets, here’ what we see.
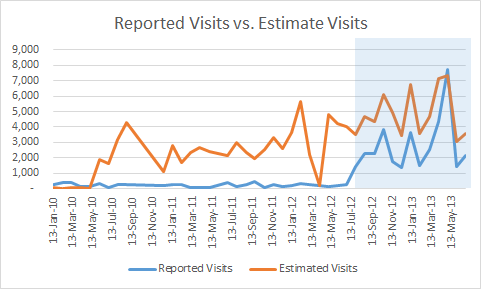
After June 2012, reported visits seems to correlate to tweet-estimated visits
Visually, there appears to be a correlation starting in July 2012. Our eyes can deceive us, though. It also appears that there is an instrumentation error in the reported data; and indeed, I found out that this data only goes back one year.
Nonetheless, let’s do a statistical calculation using Excel to see if these two datasets correlate after July 2012.
First, we do a scatter plot comparing the two datasets.
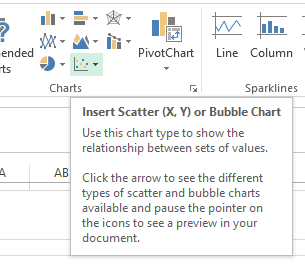
Excel’s Scatterplot Chart
Here’s what we get for that July 2012-June 2013.
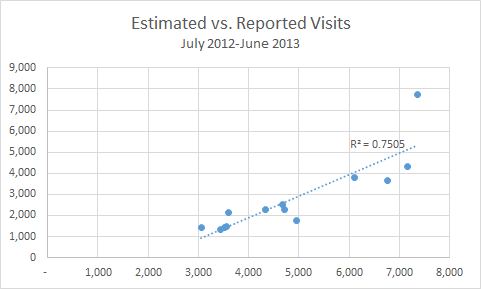
This scatterplot shows how reported visits correlate to estimated visits
This graph includes a Trendline, which Excel will calculate and draw for you. It also includes an Excel-calculated “R Squared” value. This tells us if the two datasets move together, or correlate.
An R Squared value at or near 1.0 means that the two datasets correlate. The R Squared value of 0.7505 is a strong correlation but not perfect. This gives us a 75% confidence that Tweets predict reported visits.
Looking at our graph of reported versus estimated visits, we see that our 100 tweets to 1,000 visits ratio isn’t accurate. What is the actual ratio? I calculated the mean (or average) of each data set and compared the difference. Rather than a 10:1 relationship, we get a 6:1 relationship. For each Tweet, we can expect six visits.
Here’s how this revised 6:1 dataset looks against the reported visits. This looks more reasonable and accurate.
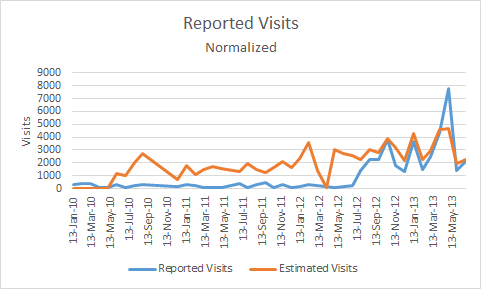
With a 6:1 tweet to visitor conversion, we see that estimated visits better match reported visits.
So, we can calculate the estimated visits for all articles by multiplying the tweets for each column by 6.38. Here’s what that graph looks like.

The visits to each of my columns over 3.62 years.
Looking at the graph, we see that the Jan 2010 through May 2010 data is fishy. So is the April 2012 article data. Did this one just suck? This graph also shows the Excel-calculated trendline and the function of that trendline.
What about comments? Do they predict readership interest and visits? Having three datasets that correlate would give us even more confidence in our data.
Here’s the scatterplot and R2 value comparing comments tweets:
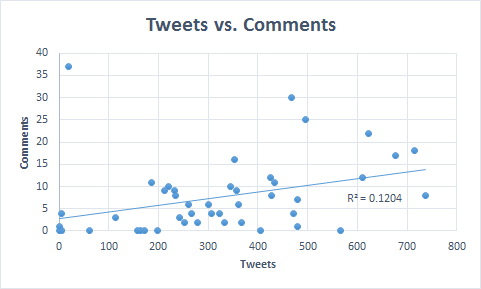
No real correlation between comments and number of tweets.
Ouch. Our R2 value is close to 0. No correlation here. This is not really surprising, so we can throw out the comments dataset for our analysis.
Real Life Application Of R2
If you want to find out how well your analytics is predicting reality, you can use this R2 value. Suppose you’re running an e-commerce site. Do a scatter plot comparing the daily sales reported by your analytics against the daily sales reported by your accounting department. This will tell you if your analytics is reporting accurately.
We have clients with correlations over 97%. This means there is a 97% likelihood that a number reported by analytics is, in fact, accurate.
You can also do an analysis of reported leads verses actual leads generated if you’re in the lead-generating business.
Drawing Conclusions From The Data
So, how have I done as an author over 3.62 years at Search Engine Land? Should you tune in?
If you look at the “Estimated Visits” graph above, you see an equation. This is the equation of the trendline.
y=2.4069x – 96343
The number snuggled up with x is the slope (also called m) of the trendline. If this slope is very positive, I’ve demonstrated a consistent increase in readership, which means I’ve done my job for Search Engine Land.
A slope of 2.4 means that each column had 2.4 more visits each day than the day before. The number -96343 is the Y intercept. Since x is measured in days, this is the number of readers I would expect to have at the beginning of time, a very negative number.
Thus, with a very positive slope, you can expect the quality of my columns to just get better and better with time (assuming visits is a proxy for quality).
What Gets Read?
An analysis of the titles of the columns gives us an idea of what kinds of titles get read.
Four of the 46 columns, or 8.7%, start with “How…”.
Thirteen of the 46 (28%) include a number, such as Five Ways To Flip Your Copywriting For Higher Conversion Rates, and 4 Anti-Science Marketing Attitudes That Keep Us In The Stone Ages. This is a bias on my part, because I know articles with such titles tend to get read more.
Only 16% are phrased as a question. Question headlines are often poorly used. They either elicit the answer “Duh” or “No, not really.” The question needs elicit answers like “Is that true?” or “How will you do that?” If your question headline generates this type of question in the mind of the reader, you’re on the right track.
A full 43% use the word “conversion.” The words “search,” “marketing,” and “landing page” appear in between 13% and 15% of titles.
I’d tell you how the top ten fared, but we would introduce a history effect error if we did. Clearly, there is an upward trend in readership over time, so readership can’t be directly attributed to the title.
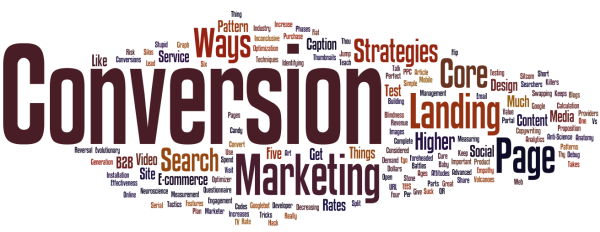
The larger words are the ones I tend to use in titles and write about.
The Final Analysis
You should follow me on Twitter if:
You want a consistently improving author (2.4 trend line slope!) who has a track record over at least 3.62 years.
Who tends to provide a finite number of “How to” steps or “Ways” to be a better marketer.
Who writes about conversion, landing pages and marketing strategies.
Who is able to work phrases into his article titles such as Flat Foreheaded, Stone Ages, Candy from a Baby, Volcano” and TV Sitcom. See below.
How can you resist?
See For Yourself
If you want to sample my work, before you follow me on Twitter, here are the top ten columns by tweets.
My Favorites
For some proof that our opinion doesn’t matter in online marketing, these are some of the columns that I thought would be big hits:
BTW…
Did I mention that you should follow me on Twitter yet?
Opmerkingen