Analytics only matters if it drives the right decisions. Here’s how we optimized our process to deliver better product analytics for higher-quality decisions.
What is product analytics?
Product analytics is the process of transforming data into insights and data-driven decisions using the collection, segmentation, and interpretation of product-level data.
4 ways to deliver better product analytics
Over the past year, the product analytics team and I have been working on four key areas that have vastly improved our ability to deliver great analytics across Intercom.
So whether you’re a seasoned leader hoping to improve the effectiveness of your existing analytics team or an individual just starting out on your own analytics journey, the following might also benefit you.
1. Foster a culture of open feedback
For analytics to inform and drive decisions that directly affect your product, there needs to be absolute trust in the analytics and the insights derived. Great analytics teams create processes to facilitate and increase that trust. Here are two ways you can do that:
Use blameless postmortems.
If one analyst is using one piece of terminology, and an engineer is using another, you’ve got a problem.
Make mistakes and slip ups an opportunity for learning. Understand why and how incidents occur but more importantly, how to prevent similar mistakes from happening again.
For example, when we notice that something has gone wrong – a broken dashboard, an issue with tracking or bad data – we fill in a postmortem doc outlining what happened, how the issue was resolved and what lessons were learned. We then hold a meeting to discuss the postmortem and brainstorm how to prevent something similar happening again.
These postmortems have resulted in concrete learnings and a number of key action items, such as introducing a new peer review process.
Create feedback loops.
Create ways to provide feedback on the queries/code of teammates. We use a peer review process based on GitHub. Any technical effort (SQL query, python code) that’s used to produce an analysis that’s shared outside of the team has to be reviewed by someone else on the team.
After a few months, we now have a central repo of reusable queries, whose quality and efficiency have greatly improved. We also have a weekly “team understanding” meeting where people are encouraged to present something they are working on in an informal way – an analysis that’s in flight, or a visualization they want input on, etc.
Allowing everyone to give open, honest, thoughtful feedback will help your team to collaborate and coach one another, and make everyone’s overall work better in terms of quality and impact.
2. Develop close partnerships
The foundation of a successful product analytics team is close partnerships with the product teams they support. There needs to be a shared understanding and context between these two worlds.
This has a lot to do with how your analytics team is organized. There are multiple ways to organize and structure an analytics team. Two popular models are centralized or embedded.
Centralized: All analysts reside in a single group that holds all the company’s analytic expertise. The analysts partner with product teams on a temporary, per project basis.
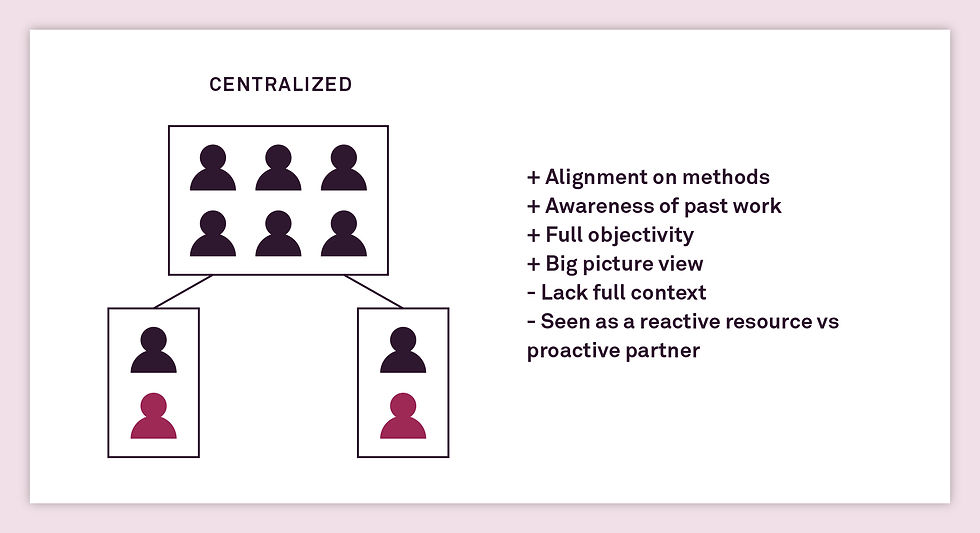
Embedded: Individual analysts are embedded within the product teams they support.
For a long time at Intercom, product analytics was completely centralized within the larger finance and analytics organisation. Analysts partnered with one or two product teams but product analytics was generally seen as more of a resource rather than a partner.
So in 2016 we moved towards a hybrid model that sits somewhere between centralized and embedded. We still have a singular product analytics team that work on foundational things together, but analysts are also embedded depending on the projects/goals at hand.
One of the smallest changes we made in this hybrid model is that analysts now attend the standups for the product teams they support. This gives them daily context into what the product teams are working on. Most importantly, it gives each analyst a sense of belonging to the product team and the product teams feel the analyst is a part of their team.
3. Use a common language
If one analyst is using one piece of terminology, and an engineer is using another, you’ve got a problem. Great analytics requires a common language so everyone’s on the same page. We did that in two ways:
Data and instrumentation
A few months ago, one of the analysts on the team drove an initiative for a new naming convention around the data we collect (“events”, as we call them). How could we design a convention that would be easily understood by non-analysts and engineers to make instrumentation straightforward, flexible and scalable?
We settled on a reusable and structured event naming model, where each event has an action, an object, a place and an owner.
We’re already seeing the benefits of this consistent language – engineers are finding the new naming structure easy to use and follow and it’s helping to add consistency and familiarity in not only the data we track but also how people talk about and think about data at Intercom.
Core metrics
There are multiple ways you can derive metrics, and many companies tend to take a purely data-driven approach. But to facilitate a shared understanding and a common language it‘s better to take a more collaborative approach.
For example, we’ve hosted a series of workshops between analytics and the product teams to talk through the core mission of our products, what behaviors they are trying to drive, and what potential signals we can extract from the data to understand if we are driving those behaviors. Using this as input, we then turn to the data to extract and analyze what core activation and engagement metrics we care about. Again this process is leading to more consistency in how people think about and talk about core metrics in Intercom.
4. Educate your organisation
Data is most powerful when it’s accessible and understood by everyone in the company. Key to that is ensuring your analytics team educate people around your organisation. There are a few simple ways you can do that. Here are two we’re trying:
Host a dedicated “office hours” each week to handle ad-hoc requests or requests from teams who you can’t provide dedicated support to.
Start a Slack channel where people in the company can come and ask data-related questions.
Helping others within the company answer key questions means more and more teams are able to make more informed decisions using data.
Of course, educating people takes some overhead early on. But we noticed that once people got the first leg-up, they started analysing data for themselves. For example, we gave a company-wide tutorial on Looker, the visualization tool we use in-house. As a result, there are a number of teams and individuals within the company who are building their own dashboards to track key metrics that they care about.
Product analytics is never over
The four strategies above will help deliver great analytics and in turn drive more effective, informed decisions at your company.
We’re constantly revisiting and tweaking how we work so that we can continually grow and evolve. If you’re building out your own analytics team I’d love to hear from you. Drop me a comment or hit me up on Twitter.
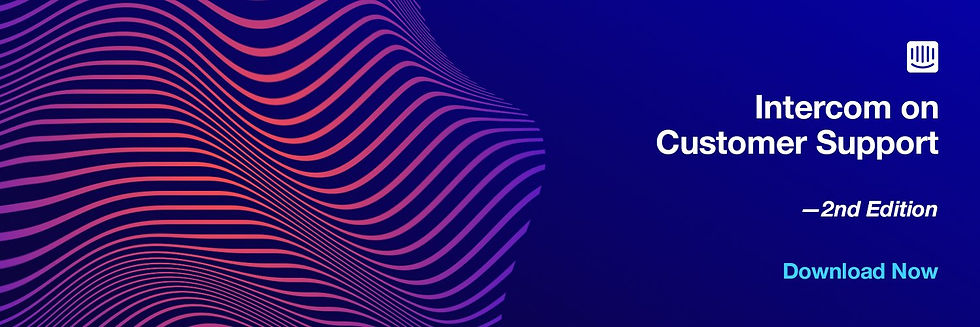
Commentaires