Analysts: Put up or shut up time!
This blog is centered around creating incredible digital experiences powered by qualitative and quantitative data insights. Every post is about unleashing the power of digital analytics (the potent combination of data, systems, software and people). But we've never stopped to consider this question:
What is the return on investment (ROI) of digital analytics? What is the incremental revenue impact on the company's bottom-line for the investment in data, systems and people?
Isn't it amazing? We've not pointed the sexy arrow of accountability on ourselves!
Let's fix that in this post. Let's calculate the ROI of digital analytics. Let's show, with real numbers (!) and a mathematical formula (oh, my!), that we are worth it!
We shall do that in in two parts.
In part one, my good friend Jesse Nichols will present his wonderful formula for computing ROA (return on analytics).
In part two, we are going to build on the formula and create a model (ok, spreadsheet :)) that you can use to compute ROA for your own company. We'll have a lot of detail in the model. It contains a sample computation you can use to build your own. It also contains multiple tabs full of specific computations of revenue incrementality delivered for various analytical efforts (Paid Search, Email Marketing, Attribution Analysis, and more). It also has one tab so full of awesomeness, you are going to have to download it to bathe in its glory.
Bottom-line: The model will give you the context you need to shine the bright sunshine of Madam Accountability on your own analytics practice.
Ready? (It is okay if you are scared. :)).
Here's part one, let me hand you over to Jesse…
______________________________________________________
Hello my dear, dear friends fighting the good fight of analytics. I felt compelled to write about this topic because I, too, am an analyst to the core. However, I've long felt the unsettling sensation of having a tremendous impact on the business, but still having to fight for attention and resources, and always wondered why that is.
After being an actual analyst for a while, I'm now managing the Google Analytics Certified Partner program, and I now realize that it wasn't just me, our entire INDUSTRY is affected by this issue.
Why is it that we analysts feel like we have some amazing untapped ability that could revolutionize any business we touch, and yet we have to fight to be included in strategic conversations where we could do the most good, and we have to fight not to be ignored when we have something important to say?
Why is it that most analytics departments are constantly under-funded and under-staffed compared to the budget-hogs in Marketing or the herds of tech workhorses in IT?
I would venture to say it’s because we’ve made an awfully poor case for the value of what we do. Businesses, by and large, don’t understand the ROI of analytics… the Return on Analytics, if you will.
Everyone else seems to get an ROI calculation, but not us. Marketing dollars (hopefully!) get measured by their Return on Ad Spend. Product improvements are quantified in incremental sales. Even internal tools are evaluated by work hours saved. Yet the analytics team rarely has its costs measured in terms of impact on The Company.
‘But we measure (and hopefully, improve!) the ROI of other things’, you say. 'The impact of analytics is the impact we have on other teams.'
Exactly! And therein lies the problem! The absolute best case scenario is that we spend all our time making everyone else look better, only to let them take all the credit.
Do we really think that if our executives believed that every dollar invested (properly) in analytics would result in ten dollars back for the company, that we would still face the massive hurdles that so many of us deal with daily? Heck no!
However, it’s true, analytics is worth at least 10x what you invest into it. These successes are ours to claim, and it’s high time we started claiming them.
So, what is ROI?
The ROI calculation is cliché and overused because it’s so simple, even a child could do it:
* How much did you invest? * How much did you make in return? * Was the latter greater than the former? (And enough so that it was worth the effort?)
With that context in mind, here’s an equation I drew up to quantify your impact as the “Return on Analytics”:
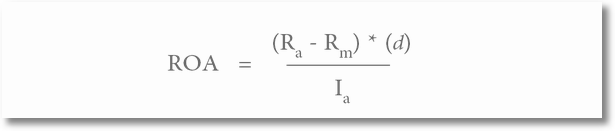
Don’t worry, its bark is worse than its bite. All it’s asking you to do is put the ROI calculation in terms of how analytics works. The formula accommodates for the critical need to compute incremental impact from deployment of an analytics practice.
You see, the challenge with analytics is that you can’t just say “how much did you make in return?” because you were (likely) already going to make something in return. So we have to figure out the impact – the incremental return that you wouldn’t have had otherwise.
So what I’ve done here is:
* Highlight the “full incremental return” within a discrete time unit (such as a day, week, month, whatever) by first subtracting the improved ROI thanks to analytics (Ra) from the original ROI that you were getting before (Rm)
* Then multiplied that impact by the duration of those time units that it will last: (d)
* Finally divide that by the costs it took to get to that impact: Ia
The end result:
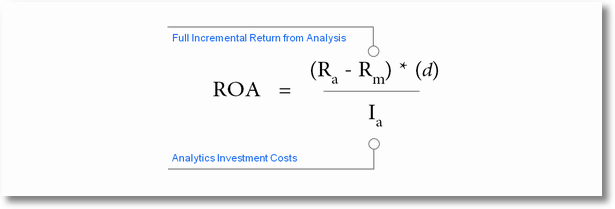
‘But it’s so much more complicated than this!’, you argue. Yes. Yes it is. But so is any computation of ROI, if you really want to be honest. What this does is to package your impact into a (relatively) easy to understand way.
Let’s take an example.
Say you’re a mid-sized company who sells hubcaps. Your digital marketing team has a monthly budget of $30k, and the company sees $120k in monthly sales from it. 400% ROI, not bad.
You then hire on an analysis ninja and pay them $5k per month to “fix” your analytics (a bargain, if you ask me), and after 6 months of data-driven improvements to campaigns & landing pages, all of a sudden the same marketing costs are bringing in $180k in sales per month, a success rate which continues on for 12 months (until a new line of hubcaps come out).
To summarize: Six months of effort, twelve months of success (/gain).
Clearly some of the credit for this goes to your marketing team. But before you jump to “my marketing is now making a 600% ROI, that’s fantastic!” and then promptly give the Marketing team more money, it is important to realize that none of this would have happened were it not for the analyst who took the holistic approach to identify the optimization opportunities.
So, let's go back to our formula (above). Punch in some of the relevant numbers and you'll see this:
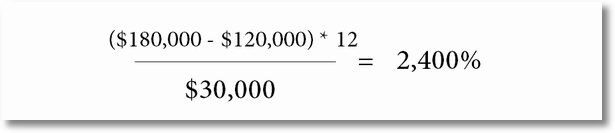
Holy guacamole! You’ve hit a gold mine! Your six month analytics driven improvement delivered twelve months of astounding results. If every dollar you’ve invested in this team paid off even half as much, then your company would be the #1 hubcap dealer in the world in no time!
This is the potential power of calculating your ROA. Attributing success where it’s due so that you can fuel the true driver of growth.
Once you’ve taken a hard look at what your investment in analytics (everything from tools to people to professional services) has produced in terms of real business results, ask where you need to invest more in order to get to a positive ROA … and not just a positive one, but the one we all imagine ourselves to be capable of.
______________________________________________________
Simple and amazing, right?
Here's the really key part… Businesses often don't understand the ROI of analytics. In fact it is not uncommon that they often don't even understand what analytics is! Here's the hidden awesomeness of computing ROA: If we can prove that there is ROI, they don't need to understand what we do as Analysts! Just like other professions (say, Accounting – what is it that they really do? :)), the analytics practice, and Analysts, will earn the right to be left alone to add value because in a very compelling way Businesses and leaders, through ROA, will know that we are adding value!!
Yes. I hear you (and Jesse acknowledged this as well).
This part of the blog post is to deliver the specificity that you've come to expect on Occam's Razor. Practical examples and specific guidance that will give you a leg up if you are convinced that bringing accountability to your analytics effort is a good thing.
The guidance is going to come to you via a customizable model in a handy dandy spreadsheet. So to speed up your ROA computation, download: Return on Analytics Calculation Model.
The model has a summary tab, a tab full of awesome specific guidance on how to compute incrementality with pitfalls and caveats, and finally a whole bunch of tabs with sample computation of incrementality across various analytical efforts.
Let's walk through the model in detail.
Tab one contains the model for an actual client from whom you can find inspiration. The first thing you'll notice is that the formula has already been created for you. No need to touch this.
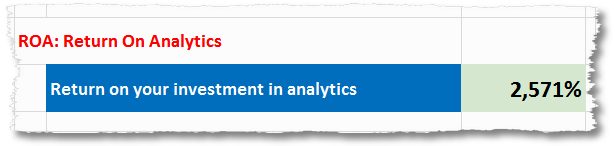
The second key element is the annual revenue for your company prior to the implementation of analytics (or a major expansion of your analytics practice). We are trying to establish a baseline. Type it in.
The third element is to calculate the total cost of ownership. Your cost! Ok, ok, you plus the hardware, software, army of consultants and BFFs. :)
Here's what you'll see for that element when you open the model…
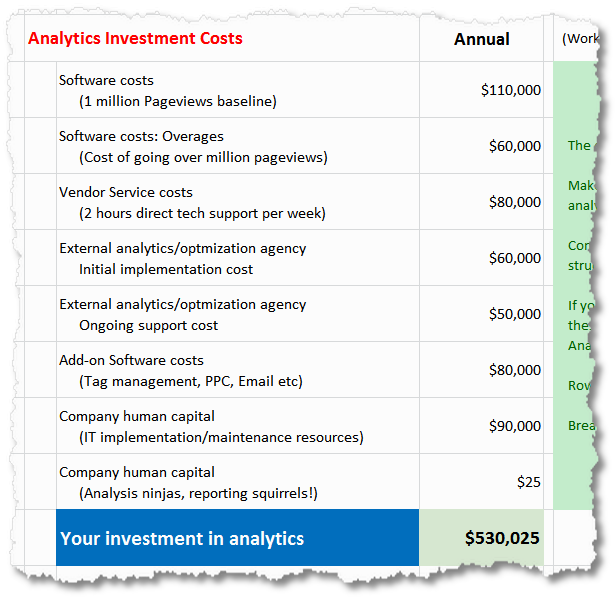
The numbers are realistic but by no means reflect what they might look like in your company. I've typed in as many things as I could think about connected to having a web analytics practice (i.e. Total Cost of Ownership).
So say you have Adobe's SiteCatalyst. You have a fixed fee you have to pay. You have a variable cost. You have a hourly support contract. You have an external agency helping you with implementation and online support. You have other software deployed, like tag management (all in vogue now and you know what, it costs money!) and specialized PPC tools and email or other software.
It also includes the important bits, ones we often overlook when creating an analytics strategy: The cost of people inside your company whose primary analytics job is implementation/IT (tagging, retagging, etc.), people whose primary job (greater than 70%) it to provide data but without insights or recommendations ("reporting squirrels," a necessary expense in any large company) and finally people whose primary job (greater than 70%) is to do analysis (and hence not data puke but provide insights and recommendations only).
And the $50,000 for IT resource and $25 for an analysis resource is just a joke I desperately hope is not true in your company (big or small, remember the 10/90 rule for incredible digital analytics success).
Not every single one of these rows will apply to your business. Say you use Yahoo! Web Analytics, the first two rows disappear, the third might not apply, but the rest might. Say you are a medium-sized company using WebTrends or Omniture, the first two rows might not be 100k/50k rather be 1,000k/350k. If you are a larger-sized company, well, you know the drill. If you are a large company you might have an army of consultants, if you are a small business that might be the free time you are getting from your cousin Ali.
So adapt the model, type in your actual costs. Calculate your digital analytics total cost of ownership. It will be revealing. I promise.
Then comes the magical part. What does your company get for all this investment?
The structure is simple, you identify the change you drove and then identify bottom-line impact of the aforementioned change after implementation of your data-influenced recommendation.
Here's what the various bits of impact look like the ROA computation model you've downloaded…

There are literally n number of things you could be driving inside your company.
In the model there are three clusters: 1. Media Optimizations 2. Content / Website Optimizations 3. Product / Company Optimization.
In each case, as you'll note above, there are examples of the type of activity that data might have informed and an example of the incremental impact.
By the way, incremental means incremental. The analytics team found an insight via their data analysis (at this moment you'll really, really regret if the primary function of your analytics practice is to data puke), that insight bundled with a specific recommendation for action was communicated effectively to the senior management, they in turn ensured it was implemented, and revenue went up.
At this point let me say something immensely important. We (Analysts) are NOT trying to claim credit for the entire uplift. We found the insight in the data and recommended an action, but many people are involved from that point on. Your marketing team went and got it implemented. Your copywriter created new copy. Your designer created new graphics. And so on and so forth. We are not trying to say here that we were singularly responsible for the incremental revenue.
We are just trying to say that that incremental revenue came from an insight produced by data analysis. So we are trying to give credit to the data. We are NOT trying to steal credit or undermine the team effort it takes to get things done in every company.
I sincerely hope that this section of the model serves as an inspiration of sorts for the vast net that data can cast in terms of driving change.
You'll see reduction of checkout abandonment rates from quantitative analysis, you'll see impact from improving task completion rate from qualitative analysis (which might drive offline conversions), you'll see impact from technical improvements, you'll see impact on the company's long-term value by improving brand perception or social media presence.
Let your mind roam wild. Look in every nook and cranny. And if your analytics practice is not focused on everything listed in this section (why not?), there is a lot of upside for you!
At least at the moment, not all the rows will apply to your business. That is ok. Fill out the ones that do. Improve over time.
Right now you are surely wondering: "Wait, what about that incremental bit? You ran over that pretty fast. That is hard stuff! "
: )
No. Did not forget that!
First, identifying incrementality is an incredibly difficult challenge. While getting perfect answers is nothing short of a life time effort, getting a good enough answer does not have to be very difficult.
So why not start there?
In the model you'll be delighted to discover a number of examples of how to compute incrementality. For example here's a screenshot of identifying incremental impact from your email marketing program.

The first thing you'll notice is that you can do this exercise in layers.
You can start with something simple. Let's say the analytics team does analysis of current email marketing metrics and identifies improvements to how your company structures the emails that go out. The recommendations are implemented and that drives an additional 100k clicks from the email campaigns. Assuming that nothing else was changed, it is now easy to measure the incremental impact of these changes.
Or maybe nothing was changed in the campaigns, but conversion rate was improved from 2% to 5% by changing the checkout conversion process for email campaigns. Well, it is easy to calculate that impact.
Or maybe you have an advanced analytics team with lots of senior management support and are able to improve the email copy and calls to action, the checkout process and do much better cross-sells and upsells and improve average order value. Well, that third cluster shows you how your computation might look.
Is it a perfect approach? Almost. Does it get you going in the right direction? Emphatically, yes!
As Voltaire put it: "Le mieux est l'ennemi du bien." (The best is the enemy of the good.)
There are other examples in the spreadsheet that should serve as guidance/inspiration for approaches you can take when you compute incrementality of the impact you deliver via your analytics practice.
Here's the section on computing value delivered by your investment in software to do multi-channel attribution modeling and the person you hired specifically to do that work…

From an impact computation perspective you can see how brutally simple the process is. Either you delivered revenue increase, or you did not.
Multi-channel attribution modeling is not easy. It has an astounding track record of failure. Identifying which model to use to attribute credit for a single conversion across multiple media channels is immensely difficult. Yet calculating whether it improved the bottom-line, whether it delivered positive ROA, is simple. You fill out the blue cells. You look at the row called Incremental Revenue. If there is something there, your digital analytics investment is worth it. If you have nothing there … well, you know … let's figure out how to say data is always worth investing in. :)
There are a few more examples I wanted to insert to really make this concrete. We cover how to compute incrementality from improving conversions, but also how to do that for the micro conversions and capture the impact of the long term impact on the business by tracking micro conversions.
Here's an excerpted version of that section…
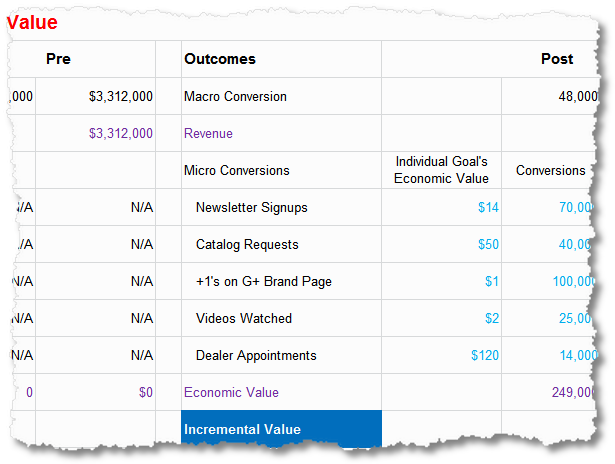
Excited? I hope so. I was giddy as a teenage school girl just creating these for the model!
There is also a tab to help you identify the incrementality from landing page optimization, and from improvements you make to the cart and checkout process. (You know my obsession with both, see best digital marketing experiences post.)
And we can't do anything related to data driven improvements without helping you compute the incrementality from insights we identify for our Paid Search campaigns.
I'll let you be delighted about both those tabs when you look at the model, and not spoil your surprise by posting images here.
The model contains one last present for you. Checkout the tab titled General Impact Analysis.
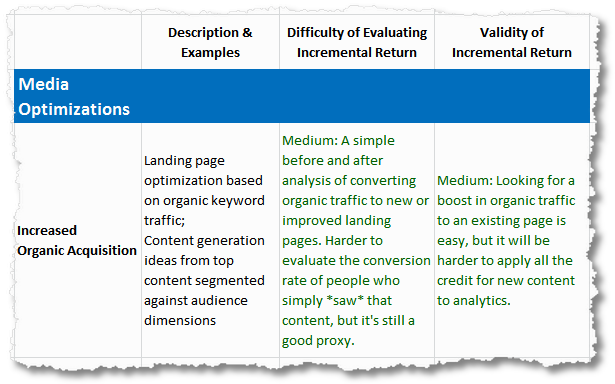
If you are new to the field you are perhaps wondering what kinds of actions you could be taking for each focus area (PPC, Email, Display, etc.). You'll find that in this tab. Column B provides description and examples of the types of outcomes you might drive in each initiative, Column D sheds light on the implementation difficulty of various types of analyses, Column E helps you understand the difficulty you'll face when computing incremental return and finally a reality check under the column titled validity of incremental return .
Here's the link again: Download: Return on Analytics Calculation Model.
Closing Thought #1: "I ain't got no incrementality!!"
It is entirely possible that at the end of looking at all the tabs in the spreadsheet you have nothing to type into the ROA computation model proposed by Jesse. A likely reason for that is that you were unable to identify any action taken as a result of your analytics practice.
There might be a simple causal factor for that. Your analytics practice is focused on DC and DR. And it turns out that you need to obsessively focus on DA for your analytics practice to have an impact on the company's bottom-line.
DC, DR & DA are three key components of any analytics practice. Data capture, data reporting, and data analysis.
I discussed this framework extensively in a recent blog post: Web Analytics Consulting: A Simple Framework For Smarter Decisions.
![web analytics consulting framework dimensional summary[1]](https://static.wixstatic.com/media/958f14_8612ac0b99064b20a659be462d439a1e~mv2.png/v1/fill/w_620,h_341,al_c,q_85,enc_auto/958f14_8612ac0b99064b20a659be462d439a1e~mv2.png)
As you'll note in the DC, DR, CA framework post, most analytics efforts (especially web analytics), consulting or in-house, are focused on collecting ever more data and in figuring out how to puke an ever-increasing amount of it in the form of standard reports via as much automation as possible. Sadly this rarely leads to the recipients gleaning any insights. Which in turn ensures that the organization is data-rich, but action-poor. Which, heartbreakingly, does result in zero actual impact on the company's bottom-line.
Hence your inability to type anything into the column titled Incremental Revenue/Impact.
So if you don't have anything to type into the various tabs in the spreadsheet I encourage you to read the DC, DR, DA post for specific guidance on what is contained in each area and how to ensure you have a better balance (egregiously focused on DA) for your analytics practice.
More investment in analytics (and your salary) will come from an ability to clearly demonstrate impact on the bottom line; otherwise, we will remain third-class citizens of the business world. The model outlined in the spreadsheet could possibly be a diagnostic tool in helping identify problems with your analytics practice (big data or small data) and figure out how to create a practice that is focused on ensuring incremental impact.
Closing Thought #2: Inspiration wrapped inside an exhortation!
You'll fail to attract investment in analytics inside your company (and a higher salary for yourself) if you are unable to show an impact on the company's bottom-line. You'll fail to show an impact on the company's bottom-line if you don't recommend actions your executives should take. You'll fail to recommend actions without an obsession on analysis of data. And yes, you'll fail to analyze data without collecting it.
If your analytics practice is not producing any actionable insights (hence no ROA) then it might be because the analytics practice is not focused on what's important to the business (advice: Biggest Mistake Web Analysts Make… And How To Avoid It!), or focused on reporting and not analysis (advice: Difference Between Reporting And Analysis), or perhaps needs a crash course in how to do better analysis (advice: Beginner's Guide To Web Data Analysis), or perhaps just needs to extract more value from the tool you have (advice: Google Analytics Tips: 10 Data Analysis Strategies That Pay Off Big! ). Identify and fix the problem. Promise me you are not going to settle for a lower salary and a boring job!
I wish you all the very best.
Before we go, my deepest thanks to Jesse Nichols for contributing to this post and inspiring a discussion that has been a long time coming.
As always, it is your turn now.
Does your company compute the incremental impact of its big data, digital analytics efforts? Is there a part of your effort that you are able to identify incremental impact for most easily? What are the biggest challenges you've faced to justify return on analytics? The model is centered on ecommerce/digital type businesses, what unique challenges do you face as a non-ecommerce/non-primarily-digital business? Do you have suggestions for improvements to Jesse's ROA formula? What are some salient hidden dangers we might be overlooking?
Please share via comments.
PS: An Ask from You: I feel that the model could use more tabs of incremental computation guidance. Can you help me create more tabs for various online or offline marketing initiatives powered by analytics? If yes, could you please create additions and email them to me? I'll be immensely grateful, and I'll add it as a tab to the model in this post (and of course credit it to you in the model, with a link to your blog / twitter profile / company). Please consider helping the community.
Thank you.
Kommentare