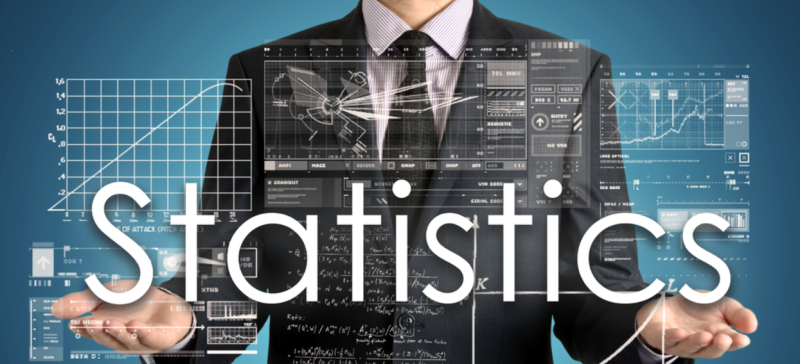
Yay, statistics!
If you run any portion of an online marketing program, you better still be reading. Marketers who don’t embrace the porcupine of statistics over the next couple of years run the risk of becoming insignificant.
If you’re still reading, I’m about to put your career or business on the fast track to statistical significance.
Let’s consider three scenarios.
Scenario One: The Failing Email List
Prim just completed sending the monthly email to her company prospect list of 3,000 people. Last month, she got 31 inquiries from the email. This month, however, she only got 20.
Sales is complaining about the low number of leads. She’s feeling the heat.
What happened? Has Prim’s email list finally been tapped out?
And what will she tell her boss?
Scenario Two: The Supercharged Landing Page
Duke’s landing page gets 20,000 visits each month like clockwork. From this traffic flow, it has been delivering 350 leads for his business each month.
One day, Duke stumbled across this column and decided he could open the sales spigot even wider by trying some different things on the site.
He made some tweaks to his landing page and re-launched it.
Shazam! He got 200 leads in first fifteen days of the month. At this rate, he’ll get 400 leads this month, one of the biggest months ever.
Duke has found a winner for sure! Or has he?
Scenario Three: The 95% Confidence Split Test
Trey completed a split test and one of his treatments has reached a magical level of confidence of 95%. The test reports a 23.49% lift in conversions! That’s big.
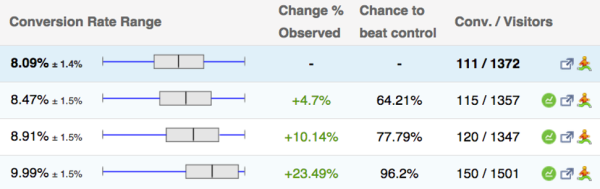
One of Trey’s split test treatments shows 23.49% increase with 96.2% confidence.
Trey tells his boss, who tells his boss, who brags about it to his wife. Trey boldly made the changes permanent on the site and then…
Crickets. No big change in his orders or revenue from the previous period.
What happened? Why will Trey’s boss’s boss have to tell his wife he’s not a winner?
Understanding Statistical Significance
In marketing, collecting data is about one thing: predicting the future. If data is a crystal ball, then we marketers are Gypsy women reading its visions.
To predict the future, we have to ask two questions:
Is the data actually telling me what happened in the past?
Is the data “good enough” to predict what will happen in the future?
For Prim and her email list, the questions are, “Was there really a significant decrease in my email response rate?” and, “Can I expect this to happen again in the following months?”
For Duke and his landing page, the questions are, “Was there really a significant increase in my leads?” and, “Can I expect the last half of this month to deliver the same results?”
For Trey and his split test, the questions are, “Did I really see a 23.49% increase in conversions?” and, “If all of my visitors see this new treatment, will more of them become leads?”
In short, we’re asking, “Can I believe what my data tells me, and, if so, will it apply to larger numbers of people or to future time periods?”
Can I Believe My Data?
The statistics we’re going to use are first going to answer the question, “Is my sample size big enough?”
Size matters in statistical significance.
There are two “sample sizes” we marketers are interested in. We want to understand if the number of impressions we generated was sufficient to predict the future, and we also want to know if the number of transactions we generated can predict the future. You can replace the word “impressions” with “visitors” or “email recipients.” You can also replace the word “transactions” with “conversions.”
Is Prim’s email list big enough to predict the future? Is 31 or 20 responses enough to tell what will happen if she sends another email?
Is 20,000 visitors and 350 conversions enough to be a crystal ball for Duke’s business?
Were there just too few visitors and conversions in Trey’s split test?
Fat Short Bells & Tall Skinny Bells
These questions can be easily answered by calculating the probability that our data is giving us an accurate conversion rate. When we graph this probability, we get what is called a bell curve. The odds of us getting a 0% or 100% conversion rate are basically zero, but Trey’s data says the odds getting a 9.99% conversion rate are high. Our graph is higher in the middle and lower on the ends.
When we graph the bell curve for any data, we can get a curve that is short and fat, or skinny and tall.
Since we have these amazing things called computers, I’m not going to take you through any of this math. I am going to show you how to understand it.
In each of our scenarios, we’re interested in the conversion rate expressed as a percentage. In Prim’s case, her conversion rate has been 31 inquiries from 3,000 emails sent. That’s a conversion rate of 1.1%. If this data predicts the future, then we would expect her next email to deliver a 1% conversion rate. However, we can see that she got 20 inquiries from her most recent email, a conversion rate of just 0.7%.
What is the probability of her getting 1% conversion rate in her next email? What is the probability of her getting 0.7% conversion rate in her next email? This is what our bell curve tells us.
The graph below estimates the probability that her next email will deliver any conversion rate between 0% and say 2%. It is most probable that she will get a 1% conversion rate. It is pretty probable that she will get a 0.7% conversion rate, too.
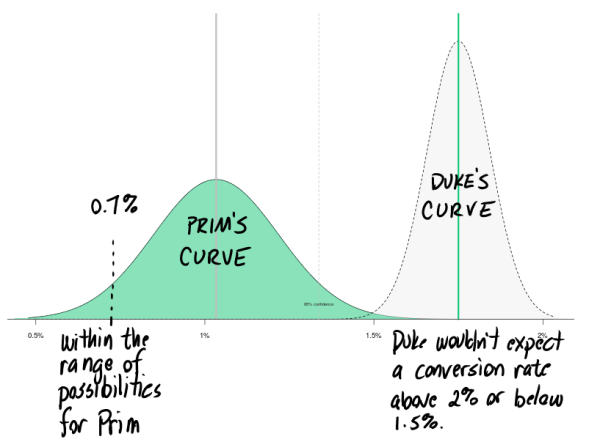
A tall, thin bell curve is better at predicting the future than a short, fat curve.
This is because her bell curve is short and fat. Her conversion rate could be all over the map from month to month. Short, fat bells come from sample sizes that are small. In her case, 31 conversions is a really small sample size. The data just can’t predict the future with a lot of accuracy.
Compare this to the graph for Duke. With 20,000 visitors and 350 conversions, his bell curve is taller and skinnier. The odds of him seeing a conversion rate below 1.5% next month is very, very small, while the odds of him repeating his 1.7% conversion rate are very high, higher than the probability of Prim seeing 1.1% again.
What do you predict the bell curve to look like for Trey? Yes, it’s shorter and fatter than Duke’s, but a little taller than Prim’s. While he has fewer impressions for each treatment, he has a higher number of conversions, making his sample size more predictive.
You should spend some time playing with the excellent tool from my good friends at Online Dialogue called the AB Test Guide Statistical Significance Calculator. It will show you how the bell curve changes when you enter different sample sizes for impressions and conversions. It expects two results, but for now just enter in the same values for the A and B scenarios.
Defining “Short” & “Thin”
There some numbers we can use to determine just how short, fat, tall or skinny our curve is, and thus our ability to tell the future.
If we have a really fat, short curve, it will have a big standard deviation. You could tell if a man was short and fat if I told you the ratio of his pant inseam to his belt size. Likewise, the standard deviation tells us something about how fat and short our curve is.
But what if a man was tall and really, really fat? He might have the same inseam-to-belt-size ratio as a short, fat man.
Standard deviation will let us calculate the span of our bell curve. Looking at Duke’s Curve above, most of his curve lies between 1.5% and 2.0%. We can calculate the range using the standard deviation.
Our tool doesn’t give us the actual confidence interval, but we can eyeball it as between 1.56% and 1.93%. All things being equal, we’d expect Duke’s conversion rate to fall between these two numbers. Anything that falls outside if this leads us to believe that something has changed; something has caused the visitors to behave differently.

The standard deviation can tell us how good our data is at predicting the future.
To understand how short and fat your curve is relative to your sample size, we calculate the standard error, which you will see with the Statistical Significance Calculator.
Whenever you use a tool that gives you graphs with a range bar, imagine the bell curve in your mind over the range bars.

The range bars in Trey’s graph match up with a bell curve.
Our tool also calculates the standard error for us. If S.E. is high, then our bell curve is shorter and fatter. For Prim, the standard error of her emails is .00185. On the other hand, Duke has a standard error of .00093, about half that of Prim. Duke has a higher chance of seeing his 1.7% conversion rate (or something close to it) next month than Prim does of seeing 1.1% again.
We will use the standard error next month when we calculate the Z-score.
What is your standard error? Calculate it and let us know in the comments.
Does A Change Mean Anything?
If we believe our sample sizes allow us to predict the future sufficiently, we can begin making changes to our pages and measuring the results. This is where statistical significance can really lead us toward making more money, or away from the rocks of bad decisions.
You are now a better marketer, but we won’t stop here. Next month we’ll find out if Prim should be nervous about her drop in email responses, deduce the odds of Duke having a big month, and explore what happened to Trey’s big win.
Challenge Question
Until next month, I have a challenge question for you. How is Prim’s sample different from Duke’s and Trey’s? Drop your answer in the comments.
Comentários